Higher rank support tensor machines for visual recognition
Article
Kotsia, I., Guo, W. and Patras, I. 2012. Higher rank support tensor machines for visual recognition. Pattern Recognition. 45 (12), pp. 4192-4203.
Type | Article |
---|---|
Title | Higher rank support tensor machines for visual recognition |
Authors | Kotsia, I., Guo, W. and Patras, I. |
Abstract | This work addresses the two class classification problem within the tensor-based large margin classification paradigm. To this end, we formulate the higher rank Support Tensor Machines (STMs), in which the parameters defining the separating hyperplane form a tensor (tensorplane) that is constrained to be the sum of rank one tensors. Subsequently, we propose two extensions in which the separating tensorplanes take into consideration the spread of the training data along the different tensor modes. More specifically, we first propose the higher rank Σ/Σw STMs that use the total or the within-class covariance matrix in order to whiten the data and thus provide invariance to affine transformations. Second, we propose the higher rank Relative Margin Support Tensor Machines (RMSTMs) that bound from above the distance of the data samples from the separating tensorplane while maximizing the margin from it. The corresponding optimization problem is solved in an iterative manner utilizing the CANDECOMP/PARAFAC (CP) decomposition, where at each iteration the parameters corresponding to the projections along a single tensor mode are estimated by solving a typical Support Vector Machine (SVM)-type optimization problem. The efficiency of the proposed method is illustrated on the problems of gait and action recognition where we report results that improve, in some cases considerably, the state of the art. |
Research Group | Research Group on Development of Intelligent Environments |
Publisher | Elsevier Science |
Journal | Pattern Recognition |
ISSN | 0031-3203 |
Publication process dates | |
Deposited | 19 Dec 2012 |
Output status | Published |
Web address (URL) | http://dx.doi.org/10.1016/j.patcog.2012.04.033 |
Language | English |
https://repository.mdx.ac.uk/item/83x07
36
total views0
total downloads0
views this month0
downloads this month
Export as
Related outputs
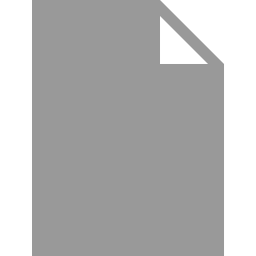