Generative recorrupted-to-recorrupted: an unsupervised image denoising network for arbitrary noise distribution
Article
Liu, Y., Wan, B., Shi, D. and Cheng, X. 2023. Generative recorrupted-to-recorrupted: an unsupervised image denoising network for arbitrary noise distribution. Remote Sensing. 15 (2). https://doi.org/10.3390/rs15020364
Type | Article |
---|---|
Title | Generative recorrupted-to-recorrupted: an unsupervised image denoising network for arbitrary noise distribution |
Authors | Liu, Y., Wan, B., Shi, D. and Cheng, X. |
Contributors | Vozel, B. |
Abstract | With the great breakthrough of supervised learning in the field of denoising, more and more works focus on end-to-end learning to train denoisers. In practice, however, it can be very challenging to obtain labels in support of this approach. The premise of this method is effective is that there is certain data support, but in practice, it is particularly difficult to obtain labels in the training data. Several unsupervised denoisers have emerged in recent years; however, to ensure their effectiveness, the noise model must be determined in advance, which limits the practical use of unsupervised denoising.n addition, obtaining inaccurate noise prior to noise estimation algorithms leads to low denoising accuracy. Therefore, we design a more practical denoiser that requires neither clean images as training labels nor noise model assumptions. Our method also needs the support of the noise model; the difference is that the model is generated by a residual image and a random mask during the network training process, and the input and target of the network are generated from a single noisy image and the noise model. At the same time, an unsupervised module and a pseudo supervised module are trained. The extensive experiments demonstrate the effectiveness of our framework and even surpass the accuracy of supervised denoising. |
Keywords | image denoising network; unsupervised; pseudo supervised |
Sustainable Development Goals | 9 Industry, innovation and infrastructure |
Middlesex University Theme | Creativity, Culture & Enterprise |
Publisher | MDPI AG |
Journal | Remote Sensing |
ISSN | |
Electronic | 2072-4292 |
Publication dates | |
Online | 06 Jan 2023 |
Jan 2023 | |
Publication process dates | |
Deposited | 03 Feb 2023 |
Submitted | 23 Nov 2022 |
Accepted | 03 Jan 2023 |
Output status | Published |
Publisher's version | License File Access Level Open |
Digital Object Identifier (DOI) | https://doi.org/10.3390/rs15020364 |
Web of Science identifier | WOS:000918765400001 |
Language | English |
https://repository.mdx.ac.uk/item/8q412
Download files
65
total views21
total downloads1
views this month0
downloads this month
Export as
Related outputs
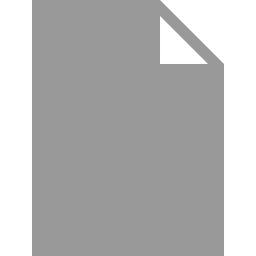
IHWC: intelligent hidden web crawler for harvesting data in urban domains
Kaur, S., Singh, A., Geetha, G. and Cheng, X. 2021. IHWC: intelligent hidden web crawler for harvesting data in urban domains. Complex & Intelligent Systems. https://doi.org/10.1007/s40747-022-00839-xA tamper-resistant broadcasting scheme for secure communication in internet of autonomous vehicles
Sun, J., Tao, J., Zhang, H., Zhao, Y., Nie, L., Cheng, X. and Zhang, T. 2023. A tamper-resistant broadcasting scheme for secure communication in internet of autonomous vehicles. IEEE Transactions on Intelligent Transportation Systems. 25 (3), pp. 2837-2846. https://doi.org/10.1109/TITS.2023.3265403Privacy-preserving and fine-grained data sharing for resource-constrained healthcare CPS devices
Bao, Y., Qiu, W. and Cheng, X. 2023. Privacy-preserving and fine-grained data sharing for resource-constrained healthcare CPS devices. Expert Systems. 40 (6). https://doi.org/10.1111/exsy.13220A novel framework for melanoma lesion segmentation using multiparallel depthwise separable and dilated convolutions with swish activations
Bukhari, M., Yasmin, S., Habib, A., Cheng, X., Ullah, F., Yoo, J. and Lee, D. 2023. A novel framework for melanoma lesion segmentation using multiparallel depthwise separable and dilated convolutions with swish activations. Journal of Healthcare Engineering. 2023 (1). https://doi.org/10.1155/2023/1847115Fake news stance detection using selective features and FakeNET
Aljrees, T., Cheng, X., Ahmed, M., Umer, M., Majeed, R., Alnowaiser, K., Abuzinadah, N. and Ashraf, I. 2023. Fake news stance detection using selective features and FakeNET. PLoS ONE. 18 (7). https://doi.org/10.1371/journal.pone.0287298PIGNUS: a deep learning model for IDS in industrial internet-of-things
Jayalaxmi, P., Saha, R., Kumar, G., Alazab, M., Conti, M. and Cheng, X. 2023. PIGNUS: a deep learning model for IDS in industrial internet-of-things. Computers and Security. 132. https://doi.org/10.1016/j.cose.2023.103315Cooperative conflict detection and resolution and safety assessment for 6G enabled unmanned aerial vehicles
Li, S., Cheng, X., Huang, X., Otaibi, S. and Wang, H. 2023. Cooperative conflict detection and resolution and safety assessment for 6G enabled unmanned aerial vehicles. IEEE Transactions on Intelligent Transportation Systems. 24 (2), pp. 2183-2198. https://doi.org/10.1109/TITS.2021.3137458A tagging SNP set method based on network community partition of linkage disequilibrium and node centrality
Wan, Q., Cheng, X., Zhang, Y., Lu, G., Wang, S. and He, S. 2022. A tagging SNP set method based on network community partition of linkage disequilibrium and node centrality. Current Bioinformatics. 17 (9), pp. 825-834. https://doi.org/10.2174/1574893617666220324155813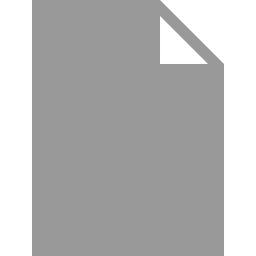
An efficient quality of services based wireless sensor network for anomaly detection using soft computing approaches
Mittal, M., Kobielnik, M., Gupta, S., Cheng, X. and Wozniak, M. 2022. An efficient quality of services based wireless sensor network for anomaly detection using soft computing approaches. Journal of Cloud Computing. 11 (1), pp. 1-21. https://doi.org/10.1186/s13677-022-00344-zA deep convolutional neural network stacked ensemble for malware threat classification in internet of things
Naeem, H., Cheng, X., Ullah, F., Jabbar, S. and Dong, S. 2022. A deep convolutional neural network stacked ensemble for malware threat classification in internet of things. Journal of Circuits, Systems and Computers. 31 (17). https://doi.org/10.1142/s0218126622503029Efficient, revocable, and privacy-preserving fine-grained data sharing with keyword search for the cloud-assisted medical IoT system
Bao, Y., Qiu, W., Tang, P. and Cheng, X. 2022. Efficient, revocable, and privacy-preserving fine-grained data sharing with keyword search for the cloud-assisted medical IoT system. IEEE Journal of Biomedical and Health Informatics. 26 (5), pp. 2041-2051. https://doi.org/10.1109/JBHI.2021.3100871Large-size data distribution in IoV based on 5G/6G compatible heterogeneous network
Yin, X., Liu, J., Cheng, X. and Xiong, X. 2022. Large-size data distribution in IoV based on 5G/6G compatible heterogeneous network. IEEE Transactions on Intelligent Transportation Systems. 25 (7), pp. 9840-9852. https://doi.org/10.1109/TITS.2021.3118701A differentiated learning environment in domain model for learning disabled learners
Thapliyal, M., Ahuja, N., Shankar, A., Cheng, X. and Kumar, M. 2022. A differentiated learning environment in domain model for learning disabled learners. Journal of Computing in Higher Education. 34, pp. 60-82. https://doi.org/10.1007/s12528-021-09278-yOffline signature verification using deep neural network with application to computer vision
Sharma, N., Gupta, S., Mehta, P., Cheng, X., Shankar, A., Singh, P. and Nayak, S. 2022. Offline signature verification using deep neural network with application to computer vision. Journal of Electronic Imaging (JEI). 31 (4). https://doi.org/10.1117/1.JEI.31.4.041210Intrusion detection and prevention system for an IoT environment
Kumar, A., Abhishek, K., Ghalib, M., Shankar, A. and Cheng, X. 2022. Intrusion detection and prevention system for an IoT environment. Digital Communications and Networks. 8 (4), pp. 540-551. https://doi.org/10.1016/j.dcan.2022.05.027An empirical study on Retinex methods for low-light image enhancement
Rasheed, M., Guo, G., Shi, D., Khan, H. and Cheng, X. 2022. An empirical study on Retinex methods for low-light image enhancement. Remote Sensing. 14 (18). https://doi.org/10.3390/rs14184608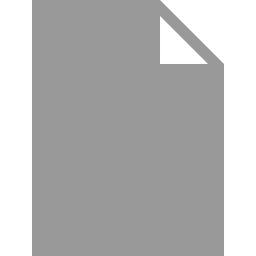
Cyber-threat detection system using a hybrid approach of transfer learning and multi-model image representation
Ullah, F., Ullah, S., Naeem, M., Mostarda, L., Rho, S. and Cheng, X. 2022. Cyber-threat detection system using a hybrid approach of transfer learning and multi-model image representation. Sensors. 22 (15), pp. 1-26. https://doi.org/10.3390/s22155883RFAP: a revocable fine-grained access control mechanism for autonomous vehicle platoon
Zhao, Y., Wang, Y., Cheng, X., Chen, H., Yu, H. and Ren, Y. 2022. RFAP: a revocable fine-grained access control mechanism for autonomous vehicle platoon. IEEE Transactions on Intelligent Transportation Systems. 23 (7), pp. 9668-9679. https://doi.org/10.1109/TITS.2021.3105458Adaptive weighted dynamic differential evolution algorithm for emergency material allocation and scheduling
Wang, T., Wu, K., Du, T. and Cheng, X. 2022. Adaptive weighted dynamic differential evolution algorithm for emergency material allocation and scheduling. Computational Intelligence. 38 (3), pp. 714-730. https://doi.org/10.1111/coin.12389CroLSSim: Cross‐language software similarity detector using hybrid approach of LSA‐based AST‐MDrep features and CNN‐LSTM model
Ullah, F., Naeem, M., Naeem, H., Cheng, X. and Alazab, M. 2022. CroLSSim: Cross‐language software similarity detector using hybrid approach of LSA‐based AST‐MDrep features and CNN‐LSTM model. International Journal of Intelligent Systems. 37 (9), pp. 5768-5795. https://doi.org/10.1002/int.22813MobiScan: an enhanced invisible screen‐camera communication system for IoT applications
Zhang, X., Liu, J., Ba, Z., Tao, Y. and Cheng, X. 2022. MobiScan: an enhanced invisible screen‐camera communication system for IoT applications. Transactions on Emerging Telecommunications Technologies. 33 (4). https://doi.org/10.1002/ett.4151Secure smart contracts for cloud-based manufacturing using Ethereum blockchain
Kumar, A., Abhishek, K., Nerurkar, P., Ghalib, M., Shankar, A. and Cheng, X. 2022. Secure smart contracts for cloud-based manufacturing using Ethereum blockchain. Transactions on Emerging Telecommunications Technologies. 33 (4). https://doi.org/10.1002/ett.4129Editorial: Security of cloud service for the manufacturing industry
Cheng, X., Liu, Z. and Ning, Y. 2022. Editorial: Security of cloud service for the manufacturing industry. Transactions on Emerging Telecommunications Technologies. 33 (4). https://doi.org/10.1002/ett.4369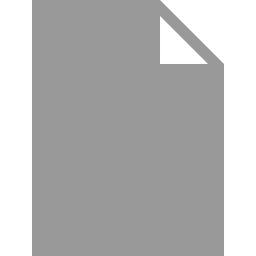
Power grid-oriented cascading failure vulnerability identifying method based on wireless sensors
Li, S., Chen, Y., Wu, X., Cheng, X. and Tian, Z. 2021. Power grid-oriented cascading failure vulnerability identifying method based on wireless sensors. Journal of Sensors. 2021, pp. 1-12. https://doi.org/10.1155/2021/8820413Secure and energy-efficient smart building architecture with emerging technology IoT
Kumar, A., Sharma, S., Goyal, N., Singh, A., Cheng, X. and Singh, P. 2021. Secure and energy-efficient smart building architecture with emerging technology IoT. Computer Communications. 176, pp. 207-217. https://doi.org/10.1016/j.comcom.2021.06.003Fuzzy decision trees embedded with evolutionary fuzzy clustering for locating users using wireless signal strength in an indoor environment
Narayanan, S., Baby, C., Perumal, B., Bhatt, R., Cheng, X., Ghalib, M. and Shankar, A. 2021. Fuzzy decision trees embedded with evolutionary fuzzy clustering for locating users using wireless signal strength in an indoor environment. International Journal of Intelligent Systems. 36 (8), pp. 4280-4267. https://doi.org/10.1002/int.22459PSSPNN: PatchShuffle Stochastic Pooling Neural Network for an explainable diagnosis of COVID-19 with multiple-way data augmentation
Wang, S., Zhang, Y., Cheng, X., Zhang, X. and Zhang, Y. 2021. PSSPNN: PatchShuffle Stochastic Pooling Neural Network for an explainable diagnosis of COVID-19 with multiple-way data augmentation. Computational and Mathematical Methods in Medicine. 2021, pp. 1-18. https://doi.org/10.1155/2021/6633755Task bundling in worker-centric mobile crowdsensing
Zhao, T., Yang, Y., Wang, E., Mumtaz, S. and Cheng, X. 2021. Task bundling in worker-centric mobile crowdsensing. International Journal of Intelligent Systems. 36 (9), pp. 4936-4961. https://doi.org/10.1002/int.22497ShadowFPE: new encrypted web application solution based on shadow DOM
Guo, X., Huang, Y., Ye, J., Yin, S., Li, M., Li, Z., Yiu, S. and Cheng, X. 2021. ShadowFPE: new encrypted web application solution based on shadow DOM. Mobile Networks and Applications. 26 (4), pp. 1733-1746. https://doi.org/10.1007/s11036-019-01509-yLearning context-aware outfit recommendation
Abugabah, A., Cheng, X. and Wang, J. 2020. Learning context-aware outfit recommendation. Symmetry. 12 (6), pp. 1-13. https://doi.org/10.3390/sym12060873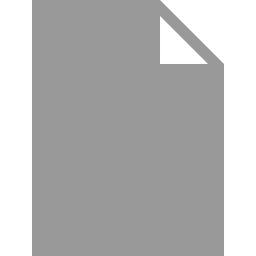