PIGNUS: a deep learning model for IDS in industrial internet-of-things
Article
Jayalaxmi, P., Saha, R., Kumar, G., Alazab, M., Conti, M. and Cheng, X. 2023. PIGNUS: a deep learning model for IDS in industrial internet-of-things. Computers and Security. 132. https://doi.org/10.1016/j.cose.2023.103315
Type | Article |
---|---|
Title | PIGNUS: a deep learning model for IDS in industrial internet-of-things |
Authors | Jayalaxmi, P., Saha, R., Kumar, G., Alazab, M., Conti, M. and Cheng, X. |
Abstract | The heterogeneous nature of the Industrial Internet of Thing (IIoT) has a considerable impact on the development of an effective Intrusion Detection System (IDS). The proliferation of linked devices results in multiple inputs from industrial sensors. IDS faces challenges in analyzing the features of the traffic and identifying anonymous behavior. Due to the unavailability of a comprehensive feature mapping method, the present IDS solutions are non-usable to identify zero-day vulnerabilities. In this paper, we introduce the first comprehensive IDS framework that combines an efficient feature-mapping technique and cascading model to solve the above-mentioned problems. We call our proposed solution deeP learnIG model intrusioN detection in indUStrial internet-of things (PIGNUS). PIGNUS integrates Auto Encoders (AE) to select optimal features and Cascade Forward Back Propagation Neural Network (CFBPNN) for classification and attack detection. The cascading model uses interconnected links from the initial layer to the output layer and determines the normal and abnormal behavior patterns and produces a perfect classification. We execute a set of experiments on five popular IIoT datasets: gas pipeline, water storage tank, NSLKDD+, UNSW-NB15, and X-IIoTID. We compare PIGNUS to the state-of-the-art models in terms of accuracy, False Positive Ratio (FPR), precision, and recall. The results show that PIGNUS provides more than accuracy, which is better on average than the existing models. In the other parameters, PIGNUS shows improved FPR, better recall, and better in precision. Overall, PIGNUS proves its efficiency as an IDS solution for IIoTs. Thus, PIGNUS is an efficient solution for IIoTs. |
Keywords | IoT; Industry; Security; Intrusion; Detection |
Sustainable Development Goals | 9 Industry, innovation and infrastructure |
Middlesex University Theme | Creativity, Culture & Enterprise |
Publisher | Elsevier |
Journal | Computers and Security |
ISSN | 0167-4048 |
Electronic | 1872-6208 |
Publication dates | |
Online | 02 Jun 2023 |
Sep 2023 | |
Publication process dates | |
Submitted | 19 Oct 2022 |
Accepted | 28 May 2023 |
Deposited | 20 Aug 2024 |
Output status | Published |
Publisher's version | License File Access Level Open |
Copyright Statement | © 2023 The Authors. Published by Elsevier Ltd. This paper is published under a Creative Commons license: https://creativecommons.org/licenses/by/4.0/ see article landing page: https://doi.org/10.1016/j.cose.2023.103315 |
Digital Object Identifier (DOI) | https://doi.org/10.1016/j.cose.2023.103315 |
Web of Science identifier | WOS:001024432400001 |
https://repository.mdx.ac.uk/item/172wyz
Download files
33
total views12
total downloads6
views this month0
downloads this month
Export as
Related outputs
Residual network-based deep learning framework for diabetic retinopathy detection
Kaushik, K., Bhardwaj, A., Cheng, X., Dahiya, S., Shankar, A., Kumar, M. and Mehrotra, T. 2025. Residual network-based deep learning framework for diabetic retinopathy detection. Journal of Database Management. 36 (1), pp. 1-21. https://doi.org/10.4018/jdm.368006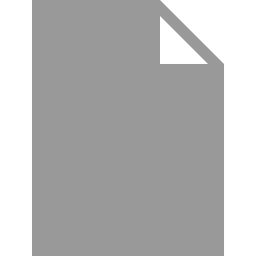
IHWC: intelligent hidden web crawler for harvesting data in urban domains
Kaur, S., Singh, A., Geetha, G. and Cheng, X. 2021. IHWC: intelligent hidden web crawler for harvesting data in urban domains. Complex & Intelligent Systems. https://doi.org/10.1007/s40747-022-00839-xA tamper-resistant broadcasting scheme for secure communication in internet of autonomous vehicles
Sun, J., Tao, J., Zhang, H., Zhao, Y., Nie, L., Cheng, X. and Zhang, T. 2023. A tamper-resistant broadcasting scheme for secure communication in internet of autonomous vehicles. IEEE Transactions on Intelligent Transportation Systems. 25 (3), pp. 2837-2846. https://doi.org/10.1109/TITS.2023.3265403Privacy-preserving and fine-grained data sharing for resource-constrained healthcare CPS devices
Bao, Y., Qiu, W. and Cheng, X. 2023. Privacy-preserving and fine-grained data sharing for resource-constrained healthcare CPS devices. Expert Systems. 40 (6). https://doi.org/10.1111/exsy.13220A novel framework for melanoma lesion segmentation using multiparallel depthwise separable and dilated convolutions with swish activations
Bukhari, M., Yasmin, S., Habib, A., Cheng, X., Ullah, F., Yoo, J. and Lee, D. 2023. A novel framework for melanoma lesion segmentation using multiparallel depthwise separable and dilated convolutions with swish activations. Journal of Healthcare Engineering. 2023 (1). https://doi.org/10.1155/2023/1847115Fake news stance detection using selective features and FakeNET
Aljrees, T., Cheng, X., Ahmed, M., Umer, M., Majeed, R., Alnowaiser, K., Abuzinadah, N. and Ashraf, I. 2023. Fake news stance detection using selective features and FakeNET. PLoS ONE. 18 (7). https://doi.org/10.1371/journal.pone.0287298Social and moral psychology of COVID-19 across 69 countries
Azevedo, F., Pavlović, T., Rêgo, G., Ay, F., Gjoneska, B., Etienne, T., Ross, R., Schönegger, P., Riaño-Moreno, J., Cichocka, A., Capraro, V., Cian, L., Longoni, C., Chan, H., Van Bavel, J., Sjåstad, H., Nezlek, J., Alfano, M., Gelfand, M., Birtel, M., Cislak, A., Lockwood, P., Abts, K., Agadullina, E., Aruta, J., Besharati, S., Bor, A., Choma, B., Crabtree, C., Cunningham, W., De, K., Ejaz, W., Elbaek, C., Findor, A., Flichtentrei, D., Franc, R., Gruber, J., Gualda, E., Horiuchi, Y., Huynh, T., Ibanez, A., Imran, M., Israelashvili, J., Jasko, K., Kantorowicz, J., Kantorowicz-Reznichenko, E., Krouwel, A., Laakasuo, M., Lamm, C., Leygue, C., Lin, M., Mansoor, M., Marie, A., Mayiwar, L., Mazepus, H., McHugh, C., Minda, J., Mitkidis, P., Olsson, A., Otterbring, T., Packer, D., Perry, A., Petersen, M., Puthillam, A., Rothmund, T., Santamaría-García, H., Schmid, P., Stoyanov, D., Tewari, S., Todosijević, B., Tsakiris, M., Tung, H., Umbres, R., Vanags, E., Vlasceanu, M., Vonasch, A., Yucel, M., Zhang, Y., Abad, M., Adler, E., Akrawi, N., Mdarhri, H., Amara, H., Amodio, D., Antazo, B., Apps, M., Ba, M., Barbosa, S., Bastian, B., Berg, A., Bernal-Zárate, M., Bernstein, M., Białek, M., Bilancini, E., Bogatyreva, N., Boncinelli, L., Booth, J., Borau, S., Buchel, O., Cameron, C., Carvalho, C., Celadin, T., Cerami, C., Chalise, H., Cheng, X., Cockcroft, K., Conway, J., Córdoba-Delgado, M., Crespi, C., Crouzevialle, M., Cutler, J., Cypryańska, M., Dabrowska, J., Daniels, M., Davis, V., Dayley, P., Delouvée, S., Denkovski, O., Dezecache, G., Dhaliwal, N., Diato, A., Di Paolo, R., Drosinou, M., Dulleck, U., Ekmanis, J., Ertan, A., Farhana, H., Farkhari, F., Farmer, H., Fenwick, A., Fidanovski, K., Flew, T., Fraser, S., Frempong, R., Fugelsang, J., Gale, J., Garcia-Navarro, E., Garladinne, P., Ghajjou, O., Gkinopoulos, T., Gray, K., Griffin, S., Gronfeldt, B., Gümren, M., Gurung, R., Halperin, E., Harris, E., Herzon, V., Hruška, M., Huang, G., Hudecek, M., Isler, O., Jangard, S., Jorgensen, F., Kachanoff, F., Kahn, J., Dangol, A., Keudel, O., Koppel, L., Koverola, M., Kubin, E., Kunnari, A., Kutiyski, Y., Laguna, O., Leota, J., Lermer, E., Levy, J., Levy, N., Li, C., Long, E., Maglić, M., McCashin, D., Metcalf, A., Mikloušić, I., El Mimouni, S., Miura, A., Molina-Paredes, J., Monroy-Fonseca, C., Morales-Marente, E., Moreau, D., Muda, R., Myer, A., Nash, K., Nesh-Nash, T., Nitschke, J., Nurse, M., Ohtsubo, Y., de Mello, V., O’Madagain, C., Onderco, M., Palacios-Galvez, M., Palomöki, J., Pan, Y., Papp, Z., Pärnamets, P., Paruzel-Czachura, M., Pavlović, Z., Payán-Gómez, C., Perander, S., Pitman, M., Prasad, R., Pyrkosz-Pacyna, J., Rathje, S., Raza, A., Rhee, K., Robertson, C., Rodríguez-Pascual, I., Saikkonen, T., Salvador-Ginez, O., Santi, G., Santiago-Tovar, N., Savage, D., Scheffer, J., Schultner, D., Schutte, E., Scott, A., Sharma, M., Sharma, P., Skali, A., Stadelmann, D., Stafford, C., Stanojević, D., Stefaniak, A., Sternisko, A., Stoica, A., Stoyanova, K., Strickland, B., Sundvall, J., Thomas, J., Tinghög, G., Torgler, B., Traast, I., Tucciarelli, R., Tyrala, M., Ungson, N., Uysal, M., Van Lange, P., van Prooijen, J., van Rooy, D., Västfjäll, D., Verkoeijen, P., Vieira, J., von Sikorski, C., Walker, A., Watermeyer, J., Wetter, E., Whillans, A., White, K., Habib, R., Willardt, R., Wohl, M., Wójcik, A., Wu, K., Yamada, Y., Yilmaz, O., Yogeeswaran, K., Ziemer, C., Zwaan, R., Boggio, P. and Sampaio, W. 2023. Social and moral psychology of COVID-19 across 69 countries. Scientific Data. 10 (1), p. 272. https://doi.org/10.1038/s41597-023-02080-8Generative recorrupted-to-recorrupted: an unsupervised image denoising network for arbitrary noise distribution
Liu, Y., Wan, B., Shi, D. and Cheng, X. 2023. Generative recorrupted-to-recorrupted: an unsupervised image denoising network for arbitrary noise distribution. Remote Sensing. 15 (2). https://doi.org/10.3390/rs15020364Cooperative conflict detection and resolution and safety assessment for 6G enabled unmanned aerial vehicles
Li, S., Cheng, X., Huang, X., Otaibi, S. and Wang, H. 2023. Cooperative conflict detection and resolution and safety assessment for 6G enabled unmanned aerial vehicles. IEEE Transactions on Intelligent Transportation Systems. 24 (2), pp. 2183-2198. https://doi.org/10.1109/TITS.2021.3137458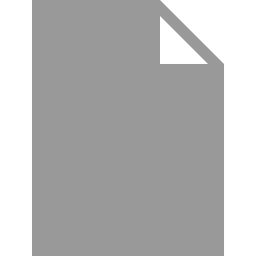
An efficient quality of services based wireless sensor network for anomaly detection using soft computing approaches
Mittal, M., Kobielnik, M., Gupta, S., Cheng, X. and Wozniak, M. 2022. An efficient quality of services based wireless sensor network for anomaly detection using soft computing approaches. Journal of Cloud Computing. 11 (1), pp. 1-21. https://doi.org/10.1186/s13677-022-00344-zEfficient, revocable, and privacy-preserving fine-grained data sharing with keyword search for the cloud-assisted medical IoT system
Bao, Y., Qiu, W., Tang, P. and Cheng, X. 2022. Efficient, revocable, and privacy-preserving fine-grained data sharing with keyword search for the cloud-assisted medical IoT system. IEEE Journal of Biomedical and Health Informatics. 26 (5), pp. 2041-2051. https://doi.org/10.1109/JBHI.2021.3100871Large-size data distribution in IoV based on 5G/6G compatible heterogeneous network
Yin, X., Liu, J., Cheng, X. and Xiong, X. 2022. Large-size data distribution in IoV based on 5G/6G compatible heterogeneous network. IEEE Transactions on Intelligent Transportation Systems. 25 (7), pp. 9840-9852. https://doi.org/10.1109/TITS.2021.3118701A differentiated learning environment in domain model for learning disabled learners
Thapliyal, M., Ahuja, N., Shankar, A., Cheng, X. and Kumar, M. 2022. A differentiated learning environment in domain model for learning disabled learners. Journal of Computing in Higher Education. 34, pp. 60-82. https://doi.org/10.1007/s12528-021-09278-yOffline signature verification using deep neural network with application to computer vision
Sharma, N., Gupta, S., Mehta, P., Cheng, X., Shankar, A., Singh, P. and Nayak, S. 2022. Offline signature verification using deep neural network with application to computer vision. Journal of Electronic Imaging (JEI). 31 (4). https://doi.org/10.1117/1.JEI.31.4.041210Intrusion detection and prevention system for an IoT environment
Kumar, A., Abhishek, K., Ghalib, M., Shankar, A. and Cheng, X. 2022. Intrusion detection and prevention system for an IoT environment. Digital Communications and Networks. 8 (4), pp. 540-551. https://doi.org/10.1016/j.dcan.2022.05.027An empirical study on Retinex methods for low-light image enhancement
Rasheed, M., Guo, G., Shi, D., Khan, H. and Cheng, X. 2022. An empirical study on Retinex methods for low-light image enhancement. Remote Sensing. 14 (18). https://doi.org/10.3390/rs14184608A tagging SNP set method based on network community partition of linkage disequilibrium and node centrality
Wan, Q., Cheng, X., Zhang, Y., Lu, G., Wang, S. and He, S. 2022. A tagging SNP set method based on network community partition of linkage disequilibrium and node centrality. Current Bioinformatics. 17 (9), pp. 825-834. https://doi.org/10.2174/1574893617666220324155813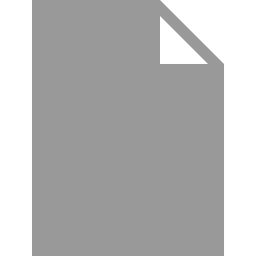
Cyber-threat detection system using a hybrid approach of transfer learning and multi-model image representation
Ullah, F., Ullah, S., Naeem, M., Mostarda, L., Rho, S. and Cheng, X. 2022. Cyber-threat detection system using a hybrid approach of transfer learning and multi-model image representation. Sensors. 22 (15), pp. 1-26. https://doi.org/10.3390/s22155883A deep convolutional neural network stacked ensemble for malware threat classification in internet of things
Naeem, H., Cheng, X., Ullah, F., Jabbar, S. and Dong, S. 2022. A deep convolutional neural network stacked ensemble for malware threat classification in internet of things. Journal of Circuits, Systems and Computers. 31 (17). https://doi.org/10.1142/s0218126622503029RFAP: a revocable fine-grained access control mechanism for autonomous vehicle platoon
Zhao, Y., Wang, Y., Cheng, X., Chen, H., Yu, H. and Ren, Y. 2022. RFAP: a revocable fine-grained access control mechanism for autonomous vehicle platoon. IEEE Transactions on Intelligent Transportation Systems. 23 (7), pp. 9668-9679. https://doi.org/10.1109/TITS.2021.3105458Predicting attitudinal and behavioral responses to COVID-19 pandemic using machine learning
Pavlović, T., Azevedo, F., De, K., Riaño-Moreno, J., Maglić, M., Gkinopoulos, T., Donnelly-Kehoe, P., Payán-Gómez, C., Huang, G., Kantorowicz, J., Birtel, M., Schönegger, P., Capraro, V., Santamaría-García, H., Yucel, M., Ibanez, A., Rathje, S., Wetter, E., Stanojević, D., van Prooijen, J., Hesse, E., Elbaek, C., Franc, R., Pavlović, Z., Mitkidis, P., Cichocka, A., Gelfand, M., Alfano, M., Ross, R., Sjåstad, H., Nezlek, J., Cislak, A., Lockwood, P., Abts, K., Agadullina, E., Amodio, D., Apps, M., Aruta, J., Besharati, S., Bor, A., Choma, B., Cunningham, W., Ejaz, W., Farmer, H., Findor, A., Gjoneska, B., Gualda, E., Huynh, T., Imran, M., Israelashvili, J., Kantorowicz-Reznichenko, E., Krouwel, A., Kutiyski, Y., Laakasuo, M., Lamm, C., Levy, J., Leygue, C., Lin, M., Mansoor, M., Marie, A., Mayiwar, L., Mazepus, H., McHugh, C., Olsson, A., Otterbring, T., Packer, D., Palomäki, J., Perry, A., Petersen, M., Puthillam, A., Rothmund, T., Schmid, P., Stadelmann, D., Stoica, A., Stoyanov, D., Stoyanova, K., Tewari, S., Todosijević, B., Torgler, B., Tsakiris, M., Tung, H., Umbreș, R., Vanags, E., Vlasceanu, M., Vonasch, A., Zhang, Y., Abad, M., Adler, E., Mdarhri, H., Antazo, B., Ay, F., Ba, M., Barbosa, S., Bastian, B., Berg, A., Białek, M., Bilancini, E., Bogatyreva, N., Boncinelli, L., Booth, J., Borau, S., Buchel, O., de Carvalho, C., Celadin, T., Cerami, C., Chalise, H., Cheng, X., Cian, L., Cockcroft, K., Conway, J., Córdoba-Delgado, M., Crespi, C., Crouzevialle, M., Cutler, J., Cypryańska, M., Dabrowska, J., Davis, V., Minda, J., Dayley, P., Delouvée, S., Denkovski, O., Dezecache, G., Dhaliwal, N., Diato, A., Di Paolo, R., Dulleck, U., Ekmanis, J., Etienne, T., Farhana, H., Farkhari, F., Fidanovski, K., Flew, T., Fraser, S., Frempong, R., Fugelsang, J., Gale, J., García-Navarro, E., Garladinne, P., Gray, K., Griffin, S., Gronfeldt, B., Gruber, J., Halperin, E., Herzon, V., Hruška, M., Hudecek, M., Isler, O., Jangard, S., Jørgensen, F., Keudel, O., Koppel, L., Koverola, M., Kunnari, A., Leota, J., Lermer, E., Li, C., Longoni, C., McCashin, D., Mikloušić, I., Molina-Paredes, J., Monroy-Fonseca, C., Morales-Marente, E., Moreau, D., Muda, R., Myer, A., Nash, K., Nitschke, J., Nurse, M., de Mello, V., Palacios-Galvez, M., Pan, Y., Papp, Z., Pärnamets, P., Paruzel-Czachura, M., Perander, S., Pitman, M., Raza, A., Rêgo, G., Robertson, C., Rodríguez-Pascual, I., Saikkonen, T., Salvador-Ginez, O., Sampaio, W., Santi, G., Schultner, D., Schutte, E., Scott, A., Skali, A., Stefaniak, A., Sternisko, A., Strickland, B., Thomas, J., Tinghög, G., Traast, I., Tucciarelli, R., Tyrala, M., Ungson, N., Uysal, M., Van Rooy, D., Västfjäll, D., Vieira, J., von Sikorski, C., Walker, A., Watermeyer, J., Willardt, R., Wohl, M., Wójcik, A., Wu, K., Yamada, Y., Yilmaz, O., Yogeeswaran, K., Ziemer, C., Zwaan, R., Boggio, P., Whillans, A., Van Lange, P., Prasad, R., Onderco, M., O'Madagain, C., Nesh-Nash, T., Laguna, O., Kubin, E., Gümren, M., Fenwick, A., Ertan, A., Bernstein, M., Amara, H. and Van Bavel, J. 2022. Predicting attitudinal and behavioral responses to COVID-19 pandemic using machine learning. PNAS Nexus. 1 (3), pp. 1-15. https://doi.org/10.1093/pnasnexus/pgac093Adaptive weighted dynamic differential evolution algorithm for emergency material allocation and scheduling
Wang, T., Wu, K., Du, T. and Cheng, X. 2022. Adaptive weighted dynamic differential evolution algorithm for emergency material allocation and scheduling. Computational Intelligence. 38 (3), pp. 714-730. https://doi.org/10.1111/coin.12389National identity predicts public health support during a global pandemic
Van Bavel, J., Cichocka, A., Capraro, V., Sjåstad, H., Nezlek, J., Pavlović, T., Alfano, M., Gelfand, M., Azevedo, F., Birtel, M., Cislak, A., Lockwood, P., Ross, R., Abts, K., Agadullina, E., Aruta, J., Besharati, S., Bor, A., Choma, B., Crabtree, C., Cunningham, W., De, K., Ejaz, W., Elbaek, C., Findor, A., Flichtentrei, D., Franc, R., Gjoneska, B., Gruber, J., Gualda, E., Horiuchi, Y., Huynh, T., Ibanez, A., Imran, M., Israelashvili, J., Jasko, K., Kantorowicz, J., Kantorowicz-Reznichenko, E., Krouwel, A., Laakasuo, M., Lamm, C., Leygue, C., Lin, M., Mansoor, M., Marie, A., Mayiwar, L., Mazepus, H., McHugh, C., Minda, J., Mitkidis, P., Olsson, A., Otterbring, T., Packer, D., Perry, A., Petersen, M., Puthillam, A., Riaño-Moreno, J., Rothmund, T., Santamaría-García, H., Schmid, P., Stoyanov, D., Tewari, S., Todosijević, B., Tsakiris, M., Tung, H., Umbreș, R., Vanags, E., Vlasceanu, M., Vonasch, A., Yucel, M., Zhang, Y., Abad, M., Adler, E., Akrawi, N., Mdarhri, H., Amara, H., Amodio, D., Antazo, B., Apps, M., Ay, F., Ba, M., Barbosa, S., Bastian, B., Berg, A., Bernal-Zárate, M., Bernstein, M., Białek, M., Bilancini, E., Bogatyreva, N., Boncinelli, L., Booth, J., Borau, S., Buchel, O., Cameron, C., Carvalho, C., Celadin, T., Cerami, C., Chalise, H., Cheng, X., Cian, L., Cockcroft, K., Conway, J., Córdoba-Delgado, M., Crespi, C., Crouzevialle, M., Cutler, J., Cypryańska, M., Dabrowska, J., Daniels, M., Davis, V., Dayley, P., Delouvee, S., Denkovski, O., Dezecache, G., Dhaliwal, N., Diato, A., Di Paolo, R., Drosinou, M., Dulleck, U., Ekmanis, J., Ertan, A., Etienne, T., Farhana, H., Farkhari, F., Farmer, H., Fenwick, A., Fidanovski, K., Flew, T., Fraser, S., Frempong, R., Fugelsang, J., Gale, J., Garcia-Navarro, E., Garladinne, P., Ghajjou, O., Gkinopoulos, T., Gray, K., Griffin, S., Gronfeldt, B., Gümren, M., Gurung, R., Halperin, E., Harris, E., Herzon, V., Hruška, M., Huang, G., Hudecek, M., Isler, O., Jangard, S., Jørgensen, F., Kachanoff, F., Kahn, J., Dangol, A., Keudel, O., Koppel, L., Koverola, M., Kubin, E., Kunnari, A., Kutiyski, Y., Laguna, O., Leota, J., Lermer, E., Levy, J., Levy, N., Li, C., Long, E., Longoni, C., Maglić, M., McCashin, D., Metcalf, A., Mikloušić, I., El Mimouni, S., Miura, A., Molina-Paredes, J., Monroy-Fonseca, C., Morales-Marente, E., Moreau, D., Muda, R., Myer, A., Nash, K., Nesh-Nash, T., Nitschke, J., Nurse, M., Ohtsubo, Y., Oldemburgo de Mello, V., O’Madagain, C., Onderco, M., Palacios-Galvez, M., Palomäki, J., Pan, Y., Papp, Z., Pärnamets, P., Paruzel-Czachura, M., Pavlović, Z., Payán-Gómez, C., Perander, S., Pitman, M., Prasad, R., Pyrkosz-Pacyna, J., Rathje, S., Raza, A., Rêgo, G., Rhee, K., Robertson, C., Rodríguez-Pascual, I., Saikkonen, T., Salvador-Ginez, O., Sampaio, W., Santi, G., Santiago-Tovar, N., Savage, D., Scheffer, J., Schönegger, P., Schultner, D., Schutte, E., Scott, A., Sharma, M., Sharma, P., Skali, A., Stadelmann, D., Stafford, C., Stanojević, D., Stefaniak, A., Sternisko, A., Stoica, A., Stoyanova, K., Strickland, B., Sundvall, J., Thomas, J., Tinghög, G., Torgler, B., Traast, I., Tucciarelli, R., Tyrala, M., Ungson, N., Uysal, M., Van Lange, P., van Prooijen, J., van Rooy, D., Västfjäll, D., Verkoeijen, P., Vieira, J., von Sikorski, C., Walker, A., Watermeyer, J., Wetter, E., Whillans, A., Willardt, R., Wohl, M., Wójcik, A., Wu, K., Yamada, Y., Yilmaz, O., Yogeeswaran, K., Ziemer, C., Zwaan, R. and Boggio, P. 2022. National identity predicts public health support during a global pandemic. Nature Communications. 13 (1), pp. 1-14. https://doi.org/10.1038/s41467-021-27668-9CroLSSim: Cross‐language software similarity detector using hybrid approach of LSA‐based AST‐MDrep features and CNN‐LSTM model
Ullah, F., Naeem, M., Naeem, H., Cheng, X. and Alazab, M. 2022. CroLSSim: Cross‐language software similarity detector using hybrid approach of LSA‐based AST‐MDrep features and CNN‐LSTM model. International Journal of Intelligent Systems. 37 (9), pp. 5768-5795. https://doi.org/10.1002/int.22813MobiScan: an enhanced invisible screen‐camera communication system for IoT applications
Zhang, X., Liu, J., Ba, Z., Tao, Y. and Cheng, X. 2022. MobiScan: an enhanced invisible screen‐camera communication system for IoT applications. Transactions on Emerging Telecommunications Technologies. 33 (4). https://doi.org/10.1002/ett.4151Secure smart contracts for cloud-based manufacturing using Ethereum blockchain
Kumar, A., Abhishek, K., Nerurkar, P., Ghalib, M., Shankar, A. and Cheng, X. 2022. Secure smart contracts for cloud-based manufacturing using Ethereum blockchain. Transactions on Emerging Telecommunications Technologies. 33 (4). https://doi.org/10.1002/ett.4129Editorial: Security of cloud service for the manufacturing industry
Cheng, X., Liu, Z. and Ning, Y. 2022. Editorial: Security of cloud service for the manufacturing industry. Transactions on Emerging Telecommunications Technologies. 33 (4). https://doi.org/10.1002/ett.4369Secure and energy-efficient smart building architecture with emerging technology IoT
Kumar, A., Sharma, S., Goyal, N., Singh, A., Cheng, X. and Singh, P. 2021. Secure and energy-efficient smart building architecture with emerging technology IoT. Computer Communications. 176, pp. 207-217. https://doi.org/10.1016/j.comcom.2021.06.003Fuzzy decision trees embedded with evolutionary fuzzy clustering for locating users using wireless signal strength in an indoor environment
Narayanan, S., Baby, C., Perumal, B., Bhatt, R., Cheng, X., Ghalib, M. and Shankar, A. 2021. Fuzzy decision trees embedded with evolutionary fuzzy clustering for locating users using wireless signal strength in an indoor environment. International Journal of Intelligent Systems. 36 (8), pp. 4280-4267. https://doi.org/10.1002/int.22459PSSPNN: PatchShuffle Stochastic Pooling Neural Network for an explainable diagnosis of COVID-19 with multiple-way data augmentation
Wang, S., Zhang, Y., Cheng, X., Zhang, X. and Zhang, Y. 2021. PSSPNN: PatchShuffle Stochastic Pooling Neural Network for an explainable diagnosis of COVID-19 with multiple-way data augmentation. Computational and Mathematical Methods in Medicine. 2021, pp. 1-18. https://doi.org/10.1155/2021/6633755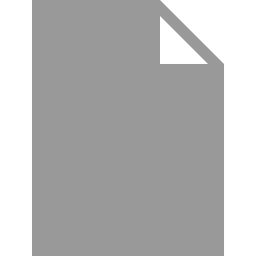
Power grid-oriented cascading failure vulnerability identifying method based on wireless sensors
Li, S., Chen, Y., Wu, X., Cheng, X. and Tian, Z. 2021. Power grid-oriented cascading failure vulnerability identifying method based on wireless sensors. Journal of Sensors. 2021, pp. 1-12. https://doi.org/10.1155/2021/8820413Task bundling in worker-centric mobile crowdsensing
Zhao, T., Yang, Y., Wang, E., Mumtaz, S. and Cheng, X. 2021. Task bundling in worker-centric mobile crowdsensing. International Journal of Intelligent Systems. 36 (9), pp. 4936-4961. https://doi.org/10.1002/int.22497ShadowFPE: new encrypted web application solution based on shadow DOM
Guo, X., Huang, Y., Ye, J., Yin, S., Li, M., Li, Z., Yiu, S. and Cheng, X. 2021. ShadowFPE: new encrypted web application solution based on shadow DOM. Mobile Networks and Applications. 26 (4), pp. 1733-1746. https://doi.org/10.1007/s11036-019-01509-yLearning context-aware outfit recommendation
Abugabah, A., Cheng, X. and Wang, J. 2020. Learning context-aware outfit recommendation. Symmetry. 12 (6), pp. 1-13. https://doi.org/10.3390/sym12060873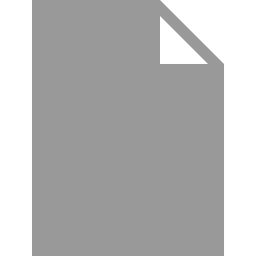