Robust continuous user authentication system using long short term memory network for healthcare
Conference paper
Tanveer, A., Lasebae, A., Ali, K., Alkhayyat, A., Ur-Rehman, M., Haq, B. and Naeem, B. 2021. Robust continuous user authentication system using long short term memory network for healthcare. Ur-Rehman, M. and Zoha, A. (ed.) 16th EAI International Conference on Body Area Networks. Glasgow, UK (Online) 25 - 26 Oct 2021 Cham Springer. https://doi.org/10.1007/978-3-030-95593-9_22
Type | Conference paper |
---|---|
Title | Robust continuous user authentication system using long short term memory network for healthcare |
Authors | Tanveer, A., Lasebae, A., Ali, K., Alkhayyat, A., Ur-Rehman, M., Haq, B. and Naeem, B. |
Abstract | A traditional user authentication method comprises of username, passwords, tokens and PINs to validate the identity of user at initial login. However, a continuous monitoring method is needed for the security of critical healthcare systems which can authenticate user on each action performed on the system in order to ensure that only legitimate user i.e., a genuine patient or medical employee is accessing the data from user account. In this aspect, the perception of employing behavioural patterns of user as biometric credential to incessantly re-verifying the user’s identity is being investigated in this research work to make the healthcare database information more secure. The keystroke behavioural biometric data represents the organisation of events in such a manner which resembles a time-series data, therefore, the recurrent neural network is used to learn the hidden and unique features of users’ behaviour saved in timeseries. Two different architectures based on per-frame classification and integrated per frame-per sequence classification are employed to assess the system performance. The proposed novel integrated model combines the notion of authenticating user on each single action and on each sequence of actions. Therefore, firstly it gives no room to imposter users to perform any illicit activity as it authenticates user on each action and secondly it tends to include the advantage of hidden unique features related to specific user saved in a sequence of actions. Hence, it identifies the abnormal user behaviour more quickly in order to escalate the security, especially in healthcare sector to secure the confidential medical data. |
Conference | 16th EAI International Conference on Body Area Networks |
Proceedings Title | Body Area Networks. Smart IoT and Big Data for Intelligent Health: 16th EAI International Conference, BODYNETS 2021, Virtual Event, October 25-26, 2021, Proceedings |
Series | Lecture Notes of the Institute for Computer Sciences, Social Informatics and Telecommunications Engineering |
Editors | Ur-Rehman, M. and Zoha, A. |
ISSN | 1867-8211 |
Electronic | 1867-822X |
ISBN | |
Paperback | 9783030955946 |
Electronic | 9783030955939 |
Publisher | Springer |
Place of publication | Cham |
Publication dates | |
Online | 10 Feb 2022 |
11 Feb 2022 | |
Publication process dates | |
Deposited | 25 Jan 2022 |
Accepted | 09 Aug 2021 |
Completed | 25 Oct 2021 |
Output status | Published |
Accepted author manuscript | File Access Level Open |
Copyright Statement | This version of the contribution has been accepted for publication, after peer review (when applicable) but is not the Version of Record and does not reflect post-acceptance improvements, or any corrections. The Version of Record is available online at: http://dx.doi.org/10.1007/978-3-030-95593-9_22. Use of this Accepted Version is subject to the publisher’s Accepted Manuscript terms of use https://www.springernature.com/gp/open-research/policies/accepted-ma... |
Digital Object Identifier (DOI) | https://doi.org/10.1007/978-3-030-95593-9_22 |
Web address (URL) of conference proceedings | https://doi.org/10.1007/978-3-030-95593-9 |
Language | English |
https://repository.mdx.ac.uk/item/89q4q
Download files
86
total views18
total downloads0
views this month1
downloads this month
Export as
Related outputs
Analyzing docker vulnerabilities through static and dynamic methods and enhancing IoT security with AWS IoT Core, CloudWatch, and GuardDuty
Ajith, V., Cyriac, T., Chavda, C., Kiyani, A.T., Chennareddy, V. and Ali, K. 2024. Analyzing docker vulnerabilities through static and dynamic methods and enhancing IoT security with AWS IoT Core, CloudWatch, and GuardDuty. IoT. 5 (3), pp. 592-607. https://doi.org/10.3390/iot5030026Energy efficiency optimisation of joint computational task offloading and resource allocation using particle swarm optimisation approach in vehicular edge networks
Alam, A., Shah, P., Trestian, R., Ali, K. and Mapp, G. 2024. Energy efficiency optimisation of joint computational task offloading and resource allocation using particle swarm optimisation approach in vehicular edge networks. Sensors. 24 (10). https://doi.org/10.3390/s24103001A novel bio-inspired bat node scheduling algorithm for dependable safety-critical wireless sensor network systems
Al-Nader, I., Lasebae, A., Raheem, R. and Ngondi, G. 2024. A novel bio-inspired bat node scheduling algorithm for dependable safety-critical wireless sensor network systems. Sensors. 24 (6). https://doi.org/10.3390/s24061928A novel scheduling algorithm for improved performance of multi-objective safety-critical WSN using spatial self-organizing feature map
Al-Nader, I., Lasebae, A. and Raheem, R. 2024. A novel scheduling algorithm for improved performance of multi-objective safety-critical WSN using spatial self-organizing feature map. Electronics. 13 (1). https://doi.org/10.3390/electronics13010019A new perceptron based neural-network algorithm to enhance the scheduling performance of safety-critical WSNs of increased dependability
Al-Nader, I, Lasebae, A. and Raheem, R. 2024. A new perceptron based neural-network algorithm to enhance the scheduling performance of safety-critical WSNs of increased dependability. So In, C., Londhe, N.D., Bhatt, N. and Kitsing, M. (ed.) 8th World Conference on Information Systems for Business Management. Bangkok, Thailand 07 - 08 Sep 2023 Springer. pp. 347-366 https://doi.org/10.1007/978-981-99-8612-5_28Information fusion-based cybersecurity threat detection for intelligent transportation system
Chowdhury, A., Naha, R., Kaisar, S., Khoshkholghi, A., Ali, K. and Galletta, A. 2023. Information fusion-based cybersecurity threat detection for intelligent transportation system. CCGridW: 4th Workshop on Secure IoT, Edge and Cloud Systems (SioTEC) 2023. Bangalore, India 01 - 04 May 2023 Bangalore, India IEEE. pp. 96-103 https://doi.org/10.1109/CCGridW59191.2023.00029Analyzing land cover and land use changes using remote sensing techniques: a temporal analysis of climate change detection with Google Earth engine
Afzal, M., Ali, K., Kasi, M., Rehman, M., Khoshkholghi, A., Haq, B. and Shah, S. 2023. Analyzing land cover and land use changes using remote sensing techniques: a temporal analysis of climate change detection with Google Earth engine. IEEE 22nd International Conference on Trust, Security and Privacy in Computing and Communications. Exeter, United Kingdom 01 - 03 Nov 2023 IEEE. pp. 2018-2023 https://doi.org/10.1109/TrustCom60117.2023.00277Joint energy and spectral optimization in Heterogeneous Vehicular Network
Alam, A., Ali, K., Trestian, R., Shah, P. and Mapp, G. 2023. Joint energy and spectral optimization in Heterogeneous Vehicular Network. Computer Networks. 238. https://doi.org/10.1016/j.comnet.2023.110111A novel scheduling algorithm for improved performance of multi-objective safety-critical wireless sensor networks using long short-term memory
Al-Nader, I., Lasebae, A., Raheem, R. and Khoshkholghi, A. 2023. A novel scheduling algorithm for improved performance of multi-objective safety-critical wireless sensor networks using long short-term memory. Electronics. 12 (23). https://doi.org/10.3390/electronics12234766Using Hidden Markov Chain for improving the dependability of safety-critical WSNs
Al-Nader, I., Lasebae, A. and Raheem, R. 2023. Using Hidden Markov Chain for improving the dependability of safety-critical WSNs. Barolli, L. (ed.) 37th International Conference on Advanced Information Networking and Applications. Federal University of Juiz de Fora, Brazil 29 - 31 Mar 2023 Cham, Switzerland. Springer. pp. 460–472 https://doi.org/10.1007/978-3-031-29056-5_40Efficient design for smart environment using Raspberry Pi with Blockchain and IoT (BRIoT)
Ponugumati, S., Ali, K., Lasebae, A., Zahoor, Z., Kiyani, A., Khoshkholghi, A. and Maddu, L. 2023. Efficient design for smart environment using Raspberry Pi with Blockchain and IoT (BRIoT). CCGridW: 4th Workshop on Secure IoT, Edge and Cloud Systems (SioTEC) 2023. Bangalore, India 01 - 04 May 2023 IEEE. pp. 75-80 https://doi.org/10.1109/CCGridW59191.2023.00026Challenges, applications and future of wireless sensors in Internet of Things: a review
Jamshed, M., Ali, K., Abbasi, Q., Imran, M. and Ur-Rehman, M. 2022. Challenges, applications and future of wireless sensors in Internet of Things: a review. IEEE Sensors Journal. https://doi.org/10.1109/JSEN.2022.3148128Adaptability of automated information systems by the development sector in developing countries: a case study
Ahmed, B., Haq, B., Ali, K. and Iqbal, M. 2021. Adaptability of automated information systems by the development sector in developing countries: a case study. International Conference on Computing, Electronic and Electrical Engineering (ICE Cube). Quetta, Pakistan 26 - 27 Oct 2021 IEEE. https://doi.org/10.1109/ICECube53880.2021.9628254Novel group handover mechanism for cooperative and coordinated mobile femtocells technology in railway environment
Raheem, R., Lasebae, A. and Raheem, A. 2022. Novel group handover mechanism for cooperative and coordinated mobile femtocells technology in railway environment. Array. 15. https://doi.org/10.1016/j.array.2022.100223Cooperative and coordinated Mobile Femtocells technology in high-speed vehicular environments: mobility and interference management
Raheem, R., Lasebae, A., Petridis, M. and Raheem, A. 2021. Cooperative and coordinated Mobile Femtocells technology in high-speed vehicular environments: mobility and interference management. Barolli, L., Woungang, I. and Enokido, T. (ed.) 35th International Conference on Advanced Information Networking and Applications (AINA-2021). Ryerson University, Toronto, Canada (Held Online) 12 - 14 May 2021 Springer. pp. 653-666 https://doi.org/10.1007/978-3-030-75100-5_56Review and implementation of resilient public safety networks: 5G, IoT and emerging technologies
Ali, K., Nguyen, H., Vien, Q., Shah, P., Raza, M., Paranthaman, V., Er-Rahmadi, B., Awais, M., Islam, S. and Rodrigues, J. 2021. Review and implementation of resilient public safety networks: 5G, IoT and emerging technologies. IEEE network. 35 (2), pp. 18-25. https://doi.org/10.1109/MNET.011.2000418Heuristic edge server placement in Industrial Internet of Things and cellular networks
Kasi, S., Kasi, M., Ali, K., Raza, M., Afzal, H., Lasebae, A., Naeem, B., Islam, S. and Rodrigues, J. 2021. Heuristic edge server placement in Industrial Internet of Things and cellular networks. IEEE Internet of Things Journal. 8 (13), pp. 10308-10317. https://doi.org/10.1109/JIOT.2020.3041805Continuous user authentication featuring keystroke dynamics based on robust recurrent confidence model and ensemble learning approach
Kiyani, A., Lasebae, A., Ali, K., Ur-Rehman, M. and Haq, B. 2020. Continuous user authentication featuring keystroke dynamics based on robust recurrent confidence model and ensemble learning approach. IEEE Access. 8, pp. 156177-156189. https://doi.org/10.1109/ACCESS.2020.3019467Deployment of drone-based small cells for public safety communication system
Ali, K., Nguyen, H., Vien, Q., Shah, P. and Raza, M. 2020. Deployment of drone-based small cells for public safety communication system. IEEE Systems Journal. 14 (2), pp. 2882-2891. https://doi.org/10.1109/JSYST.2019.2959668Architecture design for disaster resilient management network using D2D technology
Ali, K. 2019. Architecture design for disaster resilient management network using D2D technology. PhD thesis Middlesex University School of Science and TechnologyIntrusion detection and classification with autoencoded deep neural network
Rezvy, S., Petridis, M., Lasebae, A. and Zebin, T. 2019. Intrusion detection and classification with autoencoded deep neural network. Lanet, J. and Toma, C. (ed.) SecITC 2018: International Conference on Security for Information Technology and Communications. Bucharest, Romania 08 - 09 Nov 2018 Switzerland Springer Nature. https://doi.org/10.1007/978-3-030-12942-2_12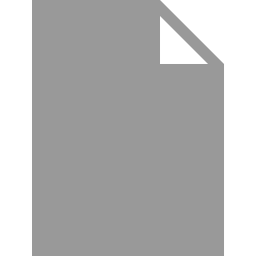
TAEO-A thermal aware & energy optimized routing protocol for wireless body area networks
Javed, M., Ahmed, G., Mahmood, D., Raza, M., Ali, K. and Ur-Rehman, M. 2019. TAEO-A thermal aware & energy optimized routing protocol for wireless body area networks. Sensors. 19 (15), pp. 1-14. https://doi.org/10.3390/s19153275An efficient deep learning model for intrusion classification and prediction in 5G and IoT networks
Rezvy, S., Luo, Y., Petridis, M., Lasebae, A. and Zebin, T. 2019. An efficient deep learning model for intrusion classification and prediction in 5G and IoT networks. 2019 53rd Annual Conference on Information Sciences and Systems (CISS). Baltimore, MD, USA, USA 20 - 22 Mar 2019 IEEE. pp. 1-6 https://doi.org/10.1109/CISS.2019.8693059The impacts of internal threats towards routing protocol for low power and lossy network performance
Le, A., Loo, J., Luo, Y. and Lasebae, A. 2013. The impacts of internal threats towards routing protocol for low power and lossy network performance. 2013 IEEE Symposium on Computers and Communications (ISCC). https://doi.org/10.1109/ISCC.2013.6755045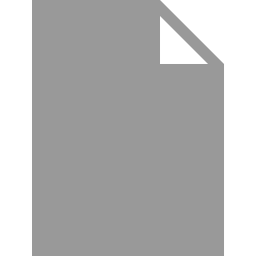