Energy efficiency optimisation of joint computational task offloading and resource allocation using particle swarm optimisation approach in vehicular edge networks
Article
Alam, A., Shah, P., Trestian, R., Ali, K. and Mapp, G. 2024. Energy efficiency optimisation of joint computational task offloading and resource allocation using particle swarm optimisation approach in vehicular edge networks. Sensors. 24 (10). https://doi.org/10.3390/s24103001
Type | Article |
---|---|
Title | Energy efficiency optimisation of joint computational task offloading and resource allocation using particle swarm optimisation approach in vehicular edge networks |
Authors | Alam, A., Shah, P., Trestian, R., Ali, K. and Mapp, G. |
Abstract | With the progression of smart vehicles, i.e., connected autonomous vehicles (CAVs), and wireless technologies, there has been an increased need for substantial computational operations for tasks such as path planning, scene recognition, and vision-based object detection. Managing these intensive computational applications is concerned with significant energy consumption. Hence, for this article, a low-cost and sustainable solution using computational offloading and efficient resource allocation at edge devices within the Internet of Vehicles (IoV) framework has been utilised. To address the quality of service (QoS) among vehicles, a trade-off between energy consumption and computational time has been taken into consideration while deciding on the offloading process and resource allocation. The offloading process has been assigned at a minimum wireless resource block level to adapt to the beyond 5G (B5G) network. The novel approach of joint optimisation of computational resources and task offloading decisions uses the meta-heuristic particle swarm optimisation (PSO) algorithm and decision analysis (DA) to find the near-optimal solution. Subsequently, a comparison is made with other proposed algorithms, namely CTORA, CODO, and Heuristics, in terms of computational efficiency and latency. The performance analysis reveals that the numerical results outperform existing algorithms, demonstrating an 8% and a 5% increase in energy efficiency. |
Keywords | energy efficiency; meta-heuristic algorithm; vehicular edge computing; particle swarm optimisation; nature-inspired algorithm; task offloading; computation resource allocation |
Sustainable Development Goals | 9 Industry, innovation and infrastructure |
Middlesex University Theme | Sustainability |
Publisher | MDPI AG |
Journal | Sensors |
ISSN | |
Electronic | 1424-8220 |
Publication dates | |
Online | 09 May 2024 |
01 May 2024 | |
Publication process dates | |
Submitted | 28 Mar 2024 |
Accepted | 08 May 2024 |
Deposited | 15 May 2024 |
Output status | Published |
Publisher's version | License File Access Level Open |
Copyright Statement | © 2024 by the authors. Licensee MDPI, Basel, Switzerland. This article is an open access article distributed under the terms and conditions of the Creative Commons Attribution (CC BY) license (https://creativecommons.org/licenses/by/4.0/). |
Digital Object Identifier (DOI) | https://doi.org/10.3390/s24103001 |
PubMed ID | 38793856 |
PubMed Central ID | PMC11125671 |
Web of Science identifier | WOS:001231651600001 |
National Library of Medicine ID | 101204366 |
https://repository.mdx.ac.uk/item/13861x
Download files
26
total views11
total downloads2
views this month0
downloads this month
Export as
Related outputs
Threshold signature in off-chain components to manage inter-chain transactions
Bigiotti, A., Mostarda, L., Navarra A, Shah, P. and Trestian, R. 2024. Threshold signature in off-chain components to manage inter-chain transactions. 6th Conference on Blockchain Research & Applications for Innovative Networks and Services. Berlin, Germany 09 - 11 Oct 2024Enhancing digital forensics readiness in big data wireless medical networks: A secure decentralised framework
Mpungu, C, George, C. and Mapp, G. 2024. Enhancing digital forensics readiness in big data wireless medical networks: A secure decentralised framework. International Journal of Network Security & Its Applications (IJNSA) . 16 (4), pp. 13-30. https://doi.org/10.5121/ijnsa.2024.16402Digital forensics readiness in big data networks: a novel framework and incident response script for Linux-Hadoop environments
Mpungu, C., George, C. and Mapp, G. 2024. Digital forensics readiness in big data networks: a novel framework and incident response script for Linux-Hadoop environments. Applied System Innovation. 7 (5). https://doi.org/10.3390/asi7050090Dissecting the hype: a study of WallStreetBets’ sentiment and network correlation on financial markets
Wang, K, Wong, B, Khoshkholghi, A., Shah, P., Naha, R, Mahanti, A and Kim, J 2024. Dissecting the hype: a study of WallStreetBets’ sentiment and network correlation on financial markets. 38th International Conference on Advanced Information Networking and Applications. Kitakyushu, Japan 17 - 19 Apr 2024 Springer. pp. 263-273 https://doi.org/10.1007/978-3-031-57853-3_22Paving the path: empowering women in STEM from university to industry
Vaka, S., Trestian, R., Baskent, C., Shayesteh, H. and Megeney, A. 2024. Paving the path: empowering women in STEM from university to industry. IEEE Global Engineering Education Conference. Kos, Greece 08 - 11 May 2024 IEEE.Building an intelligent edge environment to provide essential services in smart cities
Karthick, G., Mapp, G. and Crowcroft, J. 2023. Building an intelligent edge environment to provide essential services in smart cities. 18th Workshop on Mobility in the Evolving Internet Architecture . Madrid Spain 06 - 06 Oct 2023 New York, NY, United States Association for Computing Machinery (ACM). pp. 13-18 https://doi.org/10.1145/3615587.3615987Information fusion-based cybersecurity threat detection for intelligent transportation system
Chowdhury, A., Naha, R., Kaisar, S., Khoshkholghi, A., Ali, K. and Galletta, A. 2023. Information fusion-based cybersecurity threat detection for intelligent transportation system. CCGridW: 4th Workshop on Secure IoT, Edge and Cloud Systems (SioTEC) 2023. Bangalore, India 01 - 04 May 2023 Bangalore, India IEEE. pp. 96-103 https://doi.org/10.1109/CCGridW59191.2023.00029Improved quality of online education using prioritized multi-agent reinforcement learning for video traffic scheduling
Comsa, I., Molnar, A., Tal, I., Imhof, C., Bergamin, P., Muntean, G., Muntean, C. and Trestian, R. 2023. Improved quality of online education using prioritized multi-agent reinforcement learning for video traffic scheduling. IEEE Transactions on Broadcasting. 69 (2), pp. 436-454. https://doi.org/10.1109/TBC.2023.3246815An innovative blockchain-based traceability framework for industry 4.0 cyber-physical factory
Davis, W., Yaqoob, M., Bennett, L., Mihai, S., Hung, D., Trestian, R., Karamanoglu, M., Barn, B. and Nguyen, H. 2023. An innovative blockchain-based traceability framework for industry 4.0 cyber-physical factory. 2022 11th International Conference on Industrial Technology and Management. Oxford, United Kingdom 18 - 20 Feb 2022 New York, NY, USA Association for Computing Machinery (ACM). https://doi.org/10.1145/3588155.3588174Developing a novel digital forensics readiness framework for wireless medical networks using specialised logging
Mpungu, C., George, C. and Mapp, G. 2023. Developing a novel digital forensics readiness framework for wireless medical networks using specialised logging. Jahankhani, H. (ed.) 14th ICGS3-22: International Conference on Global Security, Safety and Sustainability. Virtual Conference 07 - 08 Sep 2022 Springer. pp. 203-226 https://doi.org/10.1007/978-3-031-20160-8_12Analyzing land cover and land use changes using remote sensing techniques: a temporal analysis of climate change detection with Google Earth engine
Afzal, M., Ali, K., Kasi, M., Rehman, M., Khoshkholghi, A., Haq, B. and Shah, S. 2023. Analyzing land cover and land use changes using remote sensing techniques: a temporal analysis of climate change detection with Google Earth engine. IEEE 22nd International Conference on Trust, Security and Privacy in Computing and Communications. Exeter, United Kingdom 01 - 03 Nov 2023 IEEE. pp. 2018-2023 https://doi.org/10.1109/TrustCom60117.2023.00277Joint energy and spectral optimization in Heterogeneous Vehicular Network
Alam, A., Ali, K., Trestian, R., Shah, P. and Mapp, G. 2023. Joint energy and spectral optimization in Heterogeneous Vehicular Network. Computer Networks. 238. https://doi.org/10.1016/j.comnet.2023.110111Efficient design for smart environment using Raspberry Pi with Blockchain and IoT (BRIoT)
Ponugumati, S., Ali, K., Lasebae, A., Zahoor, Z., Kiyani, A., Khoshkholghi, A. and Maddu, L. 2023. Efficient design for smart environment using Raspberry Pi with Blockchain and IoT (BRIoT). CCGridW: 4th Workshop on Secure IoT, Edge and Cloud Systems (SioTEC) 2023. Bangalore, India 01 - 04 May 2023 IEEE. pp. 75-80 https://doi.org/10.1109/CCGridW59191.2023.00026Mobile and wireless communications with practical use-case scenarios
Trestian, R. 2022. Mobile and wireless communications with practical use-case scenarios. CRC Press.Data-driven network performance prediction for B5G networks: a graph neural network approach
Yaqoob, M., Trestian, R. and Nguyen, H. 2022. Data-driven network performance prediction for B5G networks: a graph neural network approach. IEEE 9th International Conference on Communications and Electronics. Nha Trang City, Vietnam 27 - 29 Jul 2022 IEEE. pp. 55-60 https://doi.org/10.1109/ICCE55644.2022.9852048The Privacy Paradox - investigating people's attitude towards privacy in a time of COVID-19
Trestian, R., Celeste, E., Xie, G., Lohar, P., Bendechache, M., Brennan, R. and Tal, I. 2022. The Privacy Paradox - investigating people's attitude towards privacy in a time of COVID-19. The 14th International Conference on Communications (COMM). Bucharest (Virtual Conference) 16 - 18 Jun 2022 IEEE. https://doi.org/10.1109/COMM54429.2022.9817170Internet of Things (IoT) enabled smart navigation aid for visually impaired
Roy, M. and Shah, P. 2022. Internet of Things (IoT) enabled smart navigation aid for visually impaired. Barolli, L., Hussain, F. and Enokido, T. (ed.) Advanced Information Networking and Applications (AINA 2022). Sydney, NSW, Australia 13 - 15 Apr 2022 Springer. pp. 232-244 https://doi.org/10.1007/978-3-030-99619-2_23Exploring new traffic prediction models to build an intelligent transport system for Smart Cities
Mehta, V., Mapp, G. and Gandhi, V. 2022. Exploring new traffic prediction models to build an intelligent transport system for Smart Cities. IEEE/IFIP Network Operations and Management Symposium. Hungary 25 - 29 Apr 2022 pp. 1-6Challenges, applications and future of wireless sensors in Internet of Things: a review
Jamshed, M., Ali, K., Abbasi, Q., Imran, M. and Ur-Rehman, M. 2022. Challenges, applications and future of wireless sensors in Internet of Things: a review. IEEE Sensors Journal. https://doi.org/10.1109/JSEN.2022.3148128Adaptability of automated information systems by the development sector in developing countries: a case study
Ahmed, B., Haq, B., Ali, K. and Iqbal, M. 2021. Adaptability of automated information systems by the development sector in developing countries: a case study. International Conference on Computing, Electronic and Electrical Engineering (ICE Cube). Quetta, Pakistan 26 - 27 Oct 2021 IEEE. https://doi.org/10.1109/ICECube53880.2021.9628254Multisensory 360° videos under varying resolution levels enhance presence
Covaci, A., Saleme, E., Mesfin Assres, G., Comsa, I., Trestian, R., Santos, C. and Ghinea, G. 2023. Multisensory 360° videos under varying resolution levels enhance presence. IEEE Transactions on Visualization and Computer Graphics. 29 (4), pp. 2093-2101. https://doi.org/10.1109/TVCG.2022.3140875Digital twins: a survey on enabling technologies, challenges, trends and future prospects
Mihai, S., Yaqoob, M., Hung, D., Davis, W., Towakel, P., Raza, M., Karamanoglu, M., Barn, B., Shetve, D., Prasad, R., Venkataraman, H., Trestian, R. and Nguyen, H. 2022. Digital twins: a survey on enabling technologies, challenges, trends and future prospects. IEEE Communications Surveys and Tutorials. 24 (4), pp. 2255-2291. https://doi.org/10.1109/COMST.2022.3208773Securing future healthcare environments in a post-COVID-19 world: moving from frameworks to prototypes
Vithanwattana, N., Karthick, G., Mapp, G., George, C. and Samuels, A. 2022. Securing future healthcare environments in a post-COVID-19 world: moving from frameworks to prototypes. Journal of Reliable Intelligent Environments. 8 (3), pp. 299-315. https://doi.org/10.1007/s40860-022-00180-7RIS-aided smart manufacturing: information transmission and machine health monitoring
Hoang, T., Son, D., Barn, B., Trestian, R. and Nguyen, H. 2022. RIS-aided smart manufacturing: information transmission and machine health monitoring. IEEE Internet of Things Journal. 9 (22), pp. 22930-22943. https://doi.org/10.1109/JIOT.2022.3187189LearnSDN: optimizing routing over multimedia-based 5G-SDN using machine learning
Al-Jawad, A., Comsa, I., Shah, P. and Trestian, R. 2022. LearnSDN: optimizing routing over multimedia-based 5G-SDN using machine learning. The 14th International Conference on Communications (COMM). Bucharest, Romania [Virtual Conference] 16 - 18 Jun 2022 IEEE. https://doi.org/10.1109/COMM54429.2022.9817277Unsupervised deep learning-based reconfigurable intelligent surface aided broadcasting communications in industrial IoTs
Dinh-Van, S., Hoang, T., Trestian, R. and Nguyen, H. 2022. Unsupervised deep learning-based reconfigurable intelligent surface aided broadcasting communications in industrial IoTs. IEEE Internet of Things Journal. 9 (19), pp. 19515-19528. https://doi.org/10.1109/JIOT.2022.3169276A new privacy framework for the management of chronic diseases via mHealth in a post-Covid-19 world
Jusob, F., George, C. and Mapp, G. 2022. A new privacy framework for the management of chronic diseases via mHealth in a post-Covid-19 world. Journal of Public Health. 30 (1), pp. 37-47. https://doi.org/10.1007/s10389-021-01608-93D analytical modelling and iterative solution for high performance computing clusters
Kirsal, Y., Kirsal Ever, Y., Mapp, G. and Raza, M. 2022. 3D analytical modelling and iterative solution for high performance computing clusters. IEEE Transactions on Cloud Computing. 10 (4), pp. 2238-2251. https://doi.org/10.1109/TCC.2021.3055119Exploring a new security framework for future healthcare systems
Vithanwattana, N., Karthick, G., Mapp, G. and George, C. 2021. Exploring a new security framework for future healthcare systems. IEEE Global Communications Conference: Workshop on Securing Next-Generation Connected Healthcare Systems using Futuristic Technologies. Madrid, Spain [Hybrid: In-Person and Virtual] 07 - 11 Dec 2021 IEEE. pp. 1-6 https://doi.org/10.1109/GCWkshps52748.2021.9681967REDO: a reinforcement learning-based dynamic routing algorithm selection method for SDN
Al-Jawad, A., Comsa, I., Shah, P., Gemikonakli, O. and Trestian, R. 2021. REDO: a reinforcement learning-based dynamic routing algorithm selection method for SDN. 2021 IEEE NFV-SDN. Virtual Conference 09 - 11 Nov 2021 IEEE. pp. 54-59 https://doi.org/10.1109/NFV-SDN53031.2021.9665140A smart sensor grid to enhance irrigation techniques in Jordan using a novel event-based routing protocol
Rantisi, M., Mapp, G. and Gemikonakli, O. 2017. A smart sensor grid to enhance irrigation techniques in Jordan using a novel event-based routing protocol. Multimodal Technologies and Interaction. 1 (2). https://doi.org/10.3390/mti1020009An innovative reinforcement learning-based framework for quality of service provisioning over multimedia-based SDN environments
Al-Jawad, A., Comsa, I., Shah, P., Gemikonakli, O. and Trestian, R. 2021. An innovative reinforcement learning-based framework for quality of service provisioning over multimedia-based SDN environments. IEEE Transactions on Broadcasting. 67 (4), pp. 851-867. https://doi.org/10.1109/TBC.2021.3099728Connected vehicle testbed: development & deployment of C-ITS in the UK
Mapp, G., Ghosh, A., Paranthaman, V., Dohler, M. and Sardis, F. 2018. Connected vehicle testbed: development & deployment of C-ITS in the UK. Middlesex Repository Middlesex University.Building an Intelligent Transport Information Platform for Smart Cities
Mapp, G., Ghosh, A. and Paranthaman, V. 2017. Building an Intelligent Transport Information Platform for Smart Cities. Middlesex University Research Repository Middlesex University.PRIVATT - a closer look at people’s data privacy attitudes in times of COVID-19
Trestian, R., Xie, G., Lohar, P., Celeste, E., Bendechache, M., Brennan, R. and Tal, I. 2021. PRIVATT - a closer look at people’s data privacy attitudes in times of COVID-19. IEEE International Mediterranean Conference on Communications and Networking (MeditCom). Hybrid: In-Person in Athens, Greece and Virtual Conference 07 - 10 Sep 2021 IEEE. pp. 174-179 https://doi.org/10.1109/MeditCom49071.2021.9647539Public attitudes towards privacy in COVID-19 times in the Republic of Ireland: a pilot study
Bendechache, M., Lohar, P., Xie, G., Brennan, R., Trestian, R., Celeste, E., Kapanova, K., Jayasekera, E. and Tal, I. 2021. Public attitudes towards privacy in COVID-19 times in the Republic of Ireland: a pilot study. Information Security Journal: A Global Perspective. 30 (5), pp. 281-293. https://doi.org/10.1080/19393555.2021.1956650Privacy in a time of Covid-19: how concerned are you?
Trestian, R., Xie, G., Lohar, P., Celeste, E., Bendechache, M., Brennan, R., Jayasekera, E., Connolly, R. and Tal, I. 2021. Privacy in a time of Covid-19: how concerned are you? IEEE Security and Privacy. 19 (5), pp. 26-135. https://doi.org/10.1109/MSEC.2021.3092607Privacy in times of COVID-19: a pilot study in the Republic of Ireland
Xie, G., Lohar, P., Florea, C., Bendechache, M., Trestian, R., Brennan, R., Connolly, R. and Tal, I. 2021. Privacy in times of COVID-19: a pilot study in the Republic of Ireland. 4th Interdisciplinary Workshop on Privacy and Trust (iPAT 2021) to be held in conjunction with the 16th International Conference on Availability, Reliability and Security (ARES 2021). All-Digital Conference 17 - 20 Aug 2021 ACM. pp. 1-6 https://doi.org/10.1145/3465481.3470096Irish attitudes toward COVID tracker app & privacy: sentiment analysis on Twitter and survey data
Lohar, P., Xie, G., Bendechache, M., Brennan, R., Celeste, E., Trestian, R. and Tal, I. 2021. Irish attitudes toward COVID tracker app & privacy: sentiment analysis on Twitter and survey data. 16th International Workshop on Frontiers in Availability, Reliability and Security (FARES 2021) to be held in conjunction with the 16th International Conference on Availability, Reliability and Security (ARES 2021). All-Digital Conference 17 - 20 Aug 2021 ACM. pp. 1-8 https://doi.org/10.1145/3465481.3469193A machine learning resource allocation solution to improve video quality in remote education
Comsa, I., Molnar, A., Tal, I., Bergamin, P., Muntean, G., Muntean, C. and Trestian, R. 2021. A machine learning resource allocation solution to improve video quality in remote education. IEEE Transactions on Broadcasting. 67 (3), pp. 664-684. https://doi.org/10.1109/TBC.2021.3068872Modeling and verifying a resource allocation algorithm for secure service migration for commercial cloud systems
Karthick, G., Mapp, G., Kammueller, F. and Aiash, M. 2022. Modeling and verifying a resource allocation algorithm for secure service migration for commercial cloud systems. Computational Intelligence. 38 (3), pp. 811-828. https://doi.org/10.1111/coin.12421A digital twin framework for predictive maintenance in industry 4.0
Mihai, S., Davis, W., Hung, D., Trestian, R., Karamanoglu, M., Barn, B., Prasad, R., Venkataraman, H. and Nguyen, H. 2021. A digital twin framework for predictive maintenance in industry 4.0. HPCS 2020: 18th Annual Meeting. Barcelona, Spain (Online Virtual Conference) 22 - 27 Mar 2021 IEEE.Guaranteeing user rates with reinforcement learning in 5G radio access networks
Comsa, I., Zhang, S., Aydin, M., Kuonen, P., Trestian, R. and Ghinea, G. 2021. Guaranteeing user rates with reinforcement learning in 5G radio access networks. in: Information Resources Management Association (USA) (ed.) Research Anthology on Developing and Optimizing 5G Networks and the Impact on Society United States IGI Global. pp. 151-186Probabilistic method for time-varying reliability analysis of structure via variational bayesian neural network
Dang, H., Trestian, R., Bui-Tien, T. and Nguyen, H. 2021. Probabilistic method for time-varying reliability analysis of structure via variational bayesian neural network. Structures. 34, pp. 3703-3715. https://doi.org/10.1016/j.istruc.2021.09.069Robust continuous user authentication system using long short term memory network for healthcare
Tanveer, A., Lasebae, A., Ali, K., Alkhayyat, A., Ur-Rehman, M., Haq, B. and Naeem, B. 2021. Robust continuous user authentication system using long short term memory network for healthcare. Ur-Rehman, M. and Zoha, A. (ed.) 16th EAI International Conference on Body Area Networks. Glasgow, UK (Online) 25 - 26 Oct 2021 Cham Springer. https://doi.org/10.1007/978-3-030-95593-9_22Developing traffic predictions from source to destination using probabilistic modelling
Mehta, V., Gandhi, V. and Mapp, G. 2021. Developing traffic predictions from source to destination using probabilistic modelling. Third UK Mobile, Wearable and Ubiquitous Systems Research Symposium. Online via Zoom 05 - 06 Jul 2021Solutions for improving rich media streaming quality in heterogeneous network environments
Trestian, R. and Muntean, G. 2021. Solutions for improving rich media streaming quality in heterogeneous network environments. in: Wiley Encyclopedia of Electrical and Electronics Engineering Wiley.Review and implementation of resilient public safety networks: 5G, IoT and emerging technologies
Ali, K., Nguyen, H., Vien, Q., Shah, P., Raza, M., Paranthaman, V., Er-Rahmadi, B., Awais, M., Islam, S. and Rodrigues, J. 2021. Review and implementation of resilient public safety networks: 5G, IoT and emerging technologies. IEEE network. 35 (2), pp. 18-25. https://doi.org/10.1109/MNET.011.2000418Digital twin for 5G and beyond
Nguyen, H., Trestian, R., To, D. and Tatipamula, M. 2021. Digital twin for 5G and beyond. IEEE Communications Magazine. 59 (2), pp. 10-15. https://doi.org/10.1109/MCOM.001.2000343Heuristic edge server placement in Industrial Internet of Things and cellular networks
Kasi, S., Kasi, M., Ali, K., Raza, M., Afzal, H., Lasebae, A., Naeem, B., Islam, S. and Rodrigues, J. 2021. Heuristic edge server placement in Industrial Internet of Things and cellular networks. IEEE Internet of Things Journal. 8 (13), pp. 10308-10317. https://doi.org/10.1109/JIOT.2020.3041805Exploring analytical models for proactive resource management in highly mobile environments
Kirsal, Y., Paranthaman, V. and Mapp, G. 2018. Exploring analytical models for proactive resource management in highly mobile environments. International Journal of Computers, Communications and Control. 13 (5), pp. 837-852. https://doi.org/10.15837/ijccc.2018.5.3349The influence of human factors on 360° mulsemedia QoE
Saleme, E., Covaci, A., Assres, G., Comsa, I., Trestian, R., Santos, C.A.S. and Ghinea, G. 2021. The influence of human factors on 360° mulsemedia QoE. International Journal of Human-Computer Studies. 146. https://doi.org/10.1016/j.ijhcs.2020.102550Continuous user authentication featuring keystroke dynamics based on robust recurrent confidence model and ensemble learning approach
Kiyani, A., Lasebae, A., Ali, K., Ur-Rehman, M. and Haq, B. 2020. Continuous user authentication featuring keystroke dynamics based on robust recurrent confidence model and ensemble learning approach. IEEE Access. 8, pp. 156177-156189. https://doi.org/10.1109/ACCESS.2020.3019467From serendipity to sustainable Green IoT: technical, industrial and political perspective
Tuysuz, M. and Trestian, R. 2020. From serendipity to sustainable Green IoT: technical, industrial and political perspective. Computer Networks. 182. https://doi.org/10.1016/j.comnet.2020.107469Exploring real time traffic signalling using probabilistic approach in intelligent transport system
Mehta, V., Gandhi, V. and Mapp, G. 2018. Exploring real time traffic signalling using probabilistic approach in intelligent transport system. Mobi-UK 2018. University of Cambridge, Cambridge, UK 12 - 13 Sep 2018Exploring real time traffic signalling using probabilistic approach in intelligent transport system
Mehta, V., Gandhi, V. and Mapp, G. 2018. Exploring real time traffic signalling using probabilistic approach in intelligent transport system. 3rd CommNet2 PhD Autumn School. University of Sheffield, Sheffield, UK 17 - 19 Sep 2018Exploring a new framework to build mobile QoS-aware applications and services for future internet
Ezenwigbo, A., Kirsal, Y., Paranthaman, V., Mapp, G. and Trestian, R. 2018. Exploring a new framework to build mobile QoS-aware applications and services for future internet. Journal of Communications. 13 (10), pp. 559-573. https://doi.org/10.12720/jcm.13.10.559-573Security aspects of communications in VANETs
Mihai, S., Dokuz, N., Ali, M., Shah, P. and Trestian, R. 2020. Security aspects of communications in VANETs. COMM 2020. Bucharest, Romania 18 - 20 Jun 2020 Institute of Electrical and Electronics Engineers (IEEE). pp. 277-282 https://doi.org/10.1109/COMM48946.2020.9142034Towards autonomous driving: a machine learning-based pedestrian detection system using 16-layer LiDAR
Mihai, S., Shah, P., Mapp, G., Nguyen, H. and Trestian, R. 2020. Towards autonomous driving: a machine learning-based pedestrian detection system using 16-layer LiDAR. COMM 2020. Bucharest, Romania 18 - 20 Jun 2020 Institute of Electrical and Electronics Engineers (IEEE). pp. 271-276 https://doi.org/10.1109/COMM48946.2020.9142042Exploring the provision of reliable network storage in highly mobile environments
Ezenwigbo, A., Ramirez, J., Karthick, G., Mapp, G. and Trestian, R. 2020. Exploring the provision of reliable network storage in highly mobile environments. COMM 2020. Bucharest, Romania 18 - 20 Jun 2020 Institute of Electrical and Electronics Engineers (IEEE). pp. 255-260 https://doi.org/10.1109/COMM48946.2020.9142033A new service management framework for vehicular networks
Ramirez, J., Ezenwigbo, A., Karthick, G., Trestian, R. and Mapp, G. 2020. A new service management framework for vehicular networks. 23rd Conference on Innovation in Clouds, Internet and Networks and Workshops. Paris, France 23 - 27 Feb 2020 IEEE. pp. 162-164 https://doi.org/10.1109/ICIN48450.2020.9059441An innovative machine learning-based scheduling solution for improving live UHD video streaming quality in highly dynamic network environments
Comsa, I., Muntean, G. and Trestian, R. 2021. An innovative machine learning-based scheduling solution for improving live UHD video streaming quality in highly dynamic network environments. IEEE Transactions on Broadcasting. 67 (1), pp. 212-224. https://doi.org/10.1109/TBC.2020.2983298A digital twin framework for Industry 4.0 enabling next-gen manufacturing
Raza, M., Kumar, P., Viet Hung, D., Davis, W., Nguyen, H. and Trestian, R. 2020. A digital twin framework for Industry 4.0 enabling next-gen manufacturing. International Conference on Industrial Technology and Management (ICITM 2020). Oxford, United Kingdom 11 - 13 Feb 2020 IEEE. pp. 73-77 https://doi.org/10.1109/ICITM48982.2020.9080395Performance evaluation of routing strategies over multimedia-based SDNs under realistic environments
Al-Jawad, A., Shah, P., Gemikonakli, O., Comsa, I. and Trestian, R. 2020. Performance evaluation of routing strategies over multimedia-based SDNs under realistic environments. 6th IEEE NetSoft 2020. Virtual Conference (originally planned for Ghent, Belgium, Belgium) 29 Jun - 03 Jul 2020 Institute of Electrical and Electronics Engineers (IEEE). pp. 160-164 https://doi.org/10.1109/NetSoft48620.2020.9165521Deployment of drone-based small cells for public safety communication system
Ali, K., Nguyen, H., Vien, Q., Shah, P. and Raza, M. 2020. Deployment of drone-based small cells for public safety communication system. IEEE Systems Journal. 14 (2), pp. 2882-2891. https://doi.org/10.1109/JSYST.2019.29596685MART: A 5G SMART scheduling framework for optimizing QoS through reinforcement learning
Comsa, I., Trestian, R., Muntean, G. and Ghinea, G. 2020. 5MART: A 5G SMART scheduling framework for optimizing QoS through reinforcement learning. IEEE Transactions on Network and Service Management. 17 (2), pp. 1110-1124. https://doi.org/10.1109/TNSM.2019.2960849360° Mulsemedia: a way to improve subjective QoE in 360° videos
Covaci, A., Trestian, R., Saleme, E., Comsa, I., Assres, G., Santos, C. and Ghinea, G. 2019. 360° Mulsemedia: a way to improve subjective QoE in 360° videos. 27th ACM International Conference on Multimedia. Nice, France 21 - 25 Oct 2019 ACM. pp. 2378-2386 https://doi.org/10.1145/3343031.3350954Do I smell coffee? The tale of a 360º Mulsemedia experience
Comsa, I., Saleme, E., Covaci, A., Assres, G., Trestian, R., Santos, C. and Ghinea, G. 2020. Do I smell coffee? The tale of a 360º Mulsemedia experience. IEEE MultiMedia. 27 (1), pp. 27-36. https://doi.org/10.1109/MMUL.2019.2954405Architecture design for disaster resilient management network using D2D technology
Ali, K. 2019. Architecture design for disaster resilient management network using D2D technology. PhD thesis Middlesex University School of Science and TechnologyA comparison of reinforcement learning algorithms in fairness-oriented OFDMA schedulers
Comsa, I., Zhang, S., Aydin, M., Kuonen, P., Trestian, R. and Ghinea, G. 2019. A comparison of reinforcement learning algorithms in fairness-oriented OFDMA schedulers. Information. 10 (10). https://doi.org/10.3390/info10100315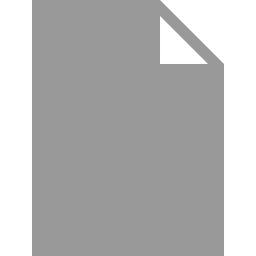
TAEO-A thermal aware & energy optimized routing protocol for wireless body area networks
Javed, M., Ahmed, G., Mahmood, D., Raza, M., Ali, K. and Ur-Rehman, M. 2019. TAEO-A thermal aware & energy optimized routing protocol for wireless body area networks. Sensors. 19 (15), pp. 1-14. https://doi.org/10.3390/s19153275Developing traffic prediction and congestion algorithms for a C-ITS network
Mehta, V., Gandhi, V. and Mapp, G. 2019. Developing traffic prediction and congestion algorithms for a C-ITS network. Second UK Mobile, Wearable and Ubiquitous Systems Research Symposium. Dept of Computer Science, University of Oxford, UK 01 Jul 2019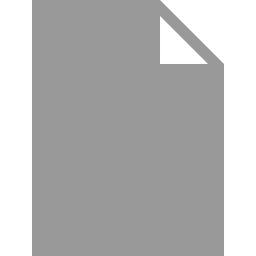