Bayesian Data-Driven approach enhances synthetic flood loss models
Article
Sairam, N., Schröter, K., Carisi, F., Wagenaar, D., Domeneghetti, A., Molinari, D., Brill, F., Priest, S., Viavattene, C., Merz, B. and Kreibich, H. 2020. Bayesian Data-Driven approach enhances synthetic flood loss models. Environmental Modelling and Software. 132. https://doi.org/10.1016/j.envsoft.2020.104798
Type | Article |
---|---|
Title | Bayesian Data-Driven approach enhances synthetic flood loss models |
Authors | Sairam, N., Schröter, K., Carisi, F., Wagenaar, D., Domeneghetti, A., Molinari, D., Brill, F., Priest, S., Viavattene, C., Merz, B. and Kreibich, H. |
Abstract | Flood loss estimation models are developed using synthetic or empirical approaches. The synthetic approach consists of what-if scenarios developed by experts. The empirical models are based on statistical analysis of empirical loss data. In this study, we propose a novel Bayesian Data-Driven approach to enhance established synthetic models using available empirical data from recorded events. For five case studies in Western Europe, the resulting Bayesian Data-Driven Synthetic (BDDS) model enhances synthetic model predictions by reducing the prediction errors and quantifying the uncertainty and reliability of loss predictions for post-event scenarios and future events. The performance of the BDDS model for a potential future event is improved by integration of empirical data once a new flood event affects the region. The BDDS model, therefore, has high potential for combining established synthetic models with local empirical loss data to provide accurate and reliable flood loss predictions for quantifying future risk. |
Research Group | Flood Hazard Research Centre |
Publisher | Elsevier |
Journal | Environmental Modelling and Software |
ISSN | 1364-8152 |
Publication dates | |
Online | 18 Jul 2020 |
01 Oct 2020 | |
Publication process dates | |
Deposited | 12 Aug 2020 |
Accepted | 10 Jul 2020 |
Output status | Published |
Accepted author manuscript | License |
Copyright Statement | © 2020. This manuscript version is made available under the CC-BY-NC-ND 4.0 license http://creativecommons.org/licenses/by-nc-nd/4.0/ |
Digital Object Identifier (DOI) | https://doi.org/10.1016/j.envsoft.2020.104798 |
Language | English |
https://repository.mdx.ac.uk/item/8908w
Download files
57
total views17
total downloads5
views this month0
downloads this month
Export as
Related outputs
Tailored flood risk communication: residents’ perspectives as starting point
Snel, K., Priest, S., Hartmann, T., Witte, P. and Geertman, S. 2024. Tailored flood risk communication: residents’ perspectives as starting point. Nature and Culture.Recognition of differences in the capacity to deal with floods—A cross-country comparison of flood risk management
Pauuw, M., Smith, G., Crabbé, A., Fournier, M., Munck af Rosenschöld, J., Priest, S. and Rekola, A. 2024. Recognition of differences in the capacity to deal with floods—A cross-country comparison of flood risk management. Journal of Flood Risk Management. https://doi.org/10.1111/jfr3.12965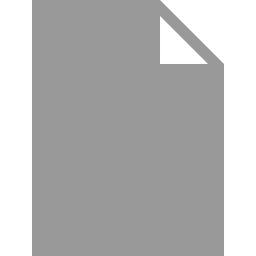
Flood risk research for improving flood risk outcomes
Priest, S. 2023. Flood risk research for improving flood risk outcomes. Journal of Flood Risk Management. 16 (1). https://doi.org/10.1111/jfr3.12888Natural flood management: Opportunities to implement nature‐based solutions on privately owned land
Thaler, T., Hudson, P., Viavattene, C. and Green, C. 2023. Natural flood management: Opportunities to implement nature‐based solutions on privately owned land. WIREs Water. 10 (3). https://doi.org/10.1002/wat2.1637On the horns of a dilemma: experts as communicators for property-level flood risk adaptation measures
Davids, P., Priest, S. and Hartmann, T. 2023. On the horns of a dilemma: experts as communicators for property-level flood risk adaptation measures. Journal of Flood Risk Management. 16 (2). https://doi.org/10.1111/jfr3.12881Wastewater monitoring for detection of public health markers during the COVID-19 pandemic: Near-source monitoring of schools in England over an academic year
Hassard, F., Vu, M., Rahimzadeh, S., Castro-Gutierrez, V., Stanton, I., Burczynska, B., Wildeboer, D., Baio, G., Brown, M., Garelick, H., Hofman, J., Kasprzyk-Hordern, B., Majeed, A., Priest, S., Denise, H., Khalifa, M., Bassano, I., Wade, M., Grimsley, J., Lundy, L., Singer, A. and Di Cesare, M. 2023. Wastewater monitoring for detection of public health markers during the COVID-19 pandemic: Near-source monitoring of schools in England over an academic year. PLoS ONE. 18 (5). https://doi.org/10.1371/journal.pone.0286259Flood and coastal erosion risk management research and development framework: working with communities
Twigger-Ross, C., Sadauskis, R., Orr, P., Jones, R., McCarthy, S., Parker, D., Priest, S. and Simms, J. 2021. Flood and coastal erosion risk management research and development framework: working with communities. Bristol, UK Environment Agency.Flood and coastal erosion risk management research and development framework: working with communities: literature review
Twigger-Ross, C., Sadauskis, R., Orr, P., Jones, R., McCarthy, S., Parker, D., Priest, S. and Simms, J. 2021. Flood and coastal erosion risk management research and development framework: working with communities: literature review. Bristol, UK Environment Agency.Cross-sectoral coordination and integration: journey planner 3
Priest, S. and Alexander, M. 2021. Cross-sectoral coordination and integration: journey planner 3. UK Environment Agency.A journey towards accountable and legitimate partnerships, Journey planner on internal partnership dynamics
Priest, S. and Alexander, M. 2021. A journey towards accountable and legitimate partnerships, Journey planner on internal partnership dynamics. Environment Agency.A journey towards accountable and legitimate partnerships, Journey planner for legitimate partnerships
Priest, S. and Alexander, M. 2021. A journey towards accountable and legitimate partnerships, Journey planner for legitimate partnerships. Environment Agency.Self-assessment framework: measuring the effectiveness of partnership governance arrangements
Alexander, M. and Priest, S. 2021. Self-assessment framework: measuring the effectiveness of partnership governance arrangements. Environment Agency.Evaluating the effectiveness of flood and coastal erosion risk governance in England and Wales
Alexander, M., Priest, S., Penning-Rowsell, E. and Cobbing, P. 2021. Evaluating the effectiveness of flood and coastal erosion risk governance in England and Wales. Bristol, UK Environment Agency.Strategic use of instruments of land policy for mobilising private land for flood risk management
Löschner, L., Hartmann, T., Priest, S. and Collentine, D. 2021. Strategic use of instruments of land policy for mobilising private land for flood risk management. Environmental Science & Policy. 118, pp. 45-48. https://doi.org/10.1016/j.envsci.2021.01.009Monitoring occurrence of SARS-CoV-2 in school populations: A wastewater-based approach
Castro-Gutierrez, V., Hassard, F., Vu, M., Leitao, R., Burczynska, B., Wildeboer, D., Stanton, I., Rahimzadeh, S., Baio, G., Garelick, H., Hofman, J., Kasprzyk-Hordern, B., Kwiatkowska, R., Majeed, A., Priest, S., Grimsley, J., Lundy, L., Singer, A. and Di Cesare, M. 2022. Monitoring occurrence of SARS-CoV-2 in school populations: A wastewater-based approach. PLoS ONE. 17 (6). https://doi.org/10.1371/journal.pone.0270168Flood risk management through a resilience lens
de Bruijn, K., Jafino, B., Merz, B., Doorn, N., Priest, S., Dahm, R., Zevenbergen, C., Aerts, J. and Comes, T. 2022. Flood risk management through a resilience lens. Communications Earth & Environment. 3 (1), pp. 1-4. https://doi.org/10.1038/s43247-022-00613-4Emergency first responders and professional wellbeing: a qualitative systematic review
Bevan, M., Priest, S., Plume, R. and Wilson, E. 2022. Emergency first responders and professional wellbeing: a qualitative systematic review. International Journal of Environmental Research and Public Health. 19 (22). https://doi.org/10.3390/ijerph192214649Foreword in: Spatial Flood Risk Management: Implementing Catchment-based Retention and Resilience on Private Land
Priest, S. 2022. Foreword in: Spatial Flood Risk Management: Implementing Catchment-based Retention and Resilience on Private Land. in: Hartmann, T., Slavíková, L. and Wilkinson, M. (ed.) Spatial Flood Risk Management: Implementing Catchment-based Retention and Resilience on Private Land Edward Elgar Publishing.Estimation of Scottish pluvial flooding Expected Annual Damages using interpolation techniques
Viavattene, C., Fadipe, D., Old, J., Thompson, V. and Thorburn, K. 2022. Estimation of Scottish pluvial flooding Expected Annual Damages using interpolation techniques. Water. 14 (3), pp. 1-17. https://doi.org/10.3390/w14030308Supporting flood and coastal erosion risk management through partnerships: key lessons
Priest, S., Alexander, M., McCarthy, S., Penning-Rowsell, E., Cumiskey, L. and Cobbing, P. 2021. Supporting flood and coastal erosion risk management through partnerships: key lessons. Bristol, UK Environment Agency.Why understanding behaviour matters for flood risk management? Journal of Flood Risk Management, 14 (2) , e12724. ISSN 1753-318X
Priest, S. 2021. Why understanding behaviour matters for flood risk management? Journal of Flood Risk Management, 14 (2) , e12724. ISSN 1753-318X. Blackwell Publishing Ltd.Needed: a systems approach to improve flood risk mitigation through private precautionary measures
Barendrecht, M., Sairam, N., Cumiskey, L., Metin, A., Holz, F., Priest, S. and Kreibich, H. 2020. Needed: a systems approach to improve flood risk mitigation through private precautionary measures. Water Security. 11. https://doi.org/10.1016/j.wasec.2020.100080Win–win for everyone? Reflecting on nature-based solutions for flood risk management from an environmental justice perspective
Kaufmann, M., Priest, S., Hudson, P., Löschner, L., Raška, P., Schindelegger, A., Slavíková, L., Stričević, R. and Vleesenbeek, T. 2021. Win–win for everyone? Reflecting on nature-based solutions for flood risk management from an environmental justice perspective. in: Ferreira, C., Kalantari, Z., Hartmann, T. and Pereira, P. (ed.) Nature-Based Solutions for Flood Mitigation: Environmental and Socio-Economic Aspects Cham Springer.'Do the resilient things.' Residents' perspectives on responsibilities for flood risk adaptation in England
Snel, K., Priest, S., Hartmann, T., Witte, P. and Geertman, S. 2021. 'Do the resilient things.' Residents' perspectives on responsibilities for flood risk adaptation in England. Journal of Flood Risk Management. 14 (3). https://doi.org/10.1111/jfr3.12727A method for monetising the mental health costs of flooding
Viavattene, C. and Priest, S. 2020. A method for monetising the mental health costs of flooding. Bristol, UK Environment Agency.Shaping flood risk governance through science-policy interfaces: Insights from England, France and The Netherlands
Hegger, D., Alexander, M., Raadgever, G., Priest, S. and Bruzzone, S. 2020. Shaping flood risk governance through science-policy interfaces: Insights from England, France and The Netherlands. Environmental Science & Policy. 106, pp. 157-165. https://doi.org/10.1016/j.envsci.2020.02.002An agenda for ethics and justice in adaptation to climate change
Byskov, M., Hyams, K., Satyal, P., Anguelovski, I., Benjamin, L., Blackburn, S., Borie, M., Caney, S., Chu, E., Edwards, G., Fourie, K., Fraser, A., Heyward, C., Jeans, H., McQuistan, C., Paavola, J., Page, E., Pelling, M., Priest, S., Swiderska, K., Tarazona, M., Thornton, T., Twigg, J. and Venn, A. 2021. An agenda for ethics and justice in adaptation to climate change. Climate and Development. 13 (1), pp. 1-9. https://doi.org/10.1080/17565529.2019.1700774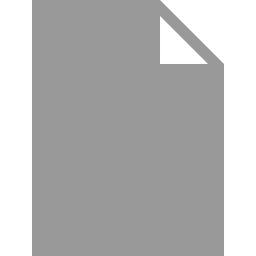